- Joined
- Aug 19, 2017
- Messages
- 2,740 (1.01/day)
On Wednesday, the MLCommons team released the MLPerf 3.0 Inference numbers, and there was an exciting submission from NVIDIA. Reportedly, NVIDIA has used software optimization to improve the already staggering performance of its latest H100 GPU by up to 54%. For reference, NVIDIA's H100 GPU first appeared on MLPerf 2.1 back in September of 2022. In just six months, NVIDIA engineers worked on AI optimizations for the MLPerf 3.0 release to find that basic software optimization can catalyze performance increases anywhere from 7-54%. The workloads for measuring the inferencing speed suite included RNN-T speech recognition, 3D U-Net medical imaging, RetinaNet object detection, ResNet-50 object classification, DLRM recommendation, and BERT 99/99.9% natural language processing.
What is interesting is that NVIDIA's submission is a bit modified. There are open and closed categories that vendors have to compete in, where closed is the mathematical equivalent of a neural network. In contrast, the open category is flexible and allows vendors to submit results based on optimizations for their hardware. The closed submission aims to provide an "apples-to-apples" hardware comparison. Given that NVIDIA opted to use the closed category, performance optimization of other vendors such as Intel and Qualcomm are not accounted for here. Still, it is interesting that optimization can lead to a performance increase of up to 54% in NVIDIA's case with its H100 GPU. Another interesting takeaway is that some comparable hardware, like Qualcomm Cloud AI 100, Intel Xeon Platinum 8480+, and NeuChips's ReccAccel N3000, failed to finish all the workloads. This is shown as "X" on the slides made by NVIDIA, stressing the need for proper ML system software support, which is NVIDIA's strength and an extensive marketing claim.
View at TechPowerUp Main Site | Source
What is interesting is that NVIDIA's submission is a bit modified. There are open and closed categories that vendors have to compete in, where closed is the mathematical equivalent of a neural network. In contrast, the open category is flexible and allows vendors to submit results based on optimizations for their hardware. The closed submission aims to provide an "apples-to-apples" hardware comparison. Given that NVIDIA opted to use the closed category, performance optimization of other vendors such as Intel and Qualcomm are not accounted for here. Still, it is interesting that optimization can lead to a performance increase of up to 54% in NVIDIA's case with its H100 GPU. Another interesting takeaway is that some comparable hardware, like Qualcomm Cloud AI 100, Intel Xeon Platinum 8480+, and NeuChips's ReccAccel N3000, failed to finish all the workloads. This is shown as "X" on the slides made by NVIDIA, stressing the need for proper ML system software support, which is NVIDIA's strength and an extensive marketing claim.
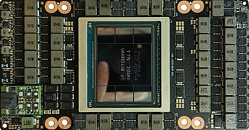
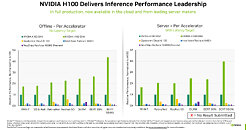
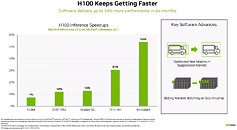
View at TechPowerUp Main Site | Source