- Joined
- Aug 19, 2017
- Messages
- 2,675 (0.99/day)
Realizing the full potential of next-generation deep learning requires highly efficient AI infrastructure. For a computing platform to be scalable and cost efficient, optimizing every layer of the AI stack, from algorithms to hardware, is essential. Advances in narrow-precision AI data formats and associated optimized algorithms have been pivotal to this journey, allowing the industry to transition from traditional 32-bit floating point precision to presently only 8 bits of precision (i.e. OCP FP8).
Narrower formats allow silicon to execute more efficient AI calculations per clock cycle, which accelerates model training and inference times. AI models take up less space, which means they require fewer data fetches from memory, and can run with better performance and efficiency. Additionally, fewer bit transfers reduces data movement over the interconnect, which can enhance application performance or cut network costs.
Bringing Together Key Industry Leaders to Set the Standard
Earlier this year, AMD, Arm, Intel, Meta, Microsoft, NVIDIA, and Qualcomm Technologies, Inc. formed the Microscaling Formats (MX) Alliance with the goal of creating and standardizing next-generation 6- and 4-bit data types for AI training and inferencing. The key enabling technology that enables sub 8-bit formats to work, referred to as microscaling, builds on a foundation of years of design space exploration and research. MX enhances the robustness and ease-of-use of existing 8-bit formats such as FP8 and INT8, thus lowering the barrier for broader adoption of single digit bit training and inference.
The initial MX specification introduces four concrete floating point and interger-based data formats (MXFP8, MXFP6, MXFP4, and MXINT8) that are compatible with current AI stacks, upport implementation flexibility across both hardware and software, and enable finegrain microscaling at the hardware level. Extensive studies demonstrate that MX formats can be easily deployed for many diverse real-world cases such as large language models, computer vision, and recommender systems. My technology also enables LLM pre-training at 6- and 4-bit precisions without any modifications to conventional training recipes.
Democratizing AI Capabilities
In the evolving landscape of AI, open standards are critical to foster innovation, collaboration, and widespread adoption. These standards offer a unifying framework that enables consistent toolchains, model development, and interoperability across the AI ecosystem. This further empowers developers and organizations to harness the full potential of AI while mitigating the fragmentation and technology constraints that could otherwise stifle progress.
In this spirit, the MX Alliance has released the Microscaling Formats (MX) Specification v1.0 in an open, license-free format through the Open Compute Project Foundation (OCP) to enable and encourage broad industry adoption and provide the foundation for potential future narrow-format innovations. Additionally, a white paper and emulation libraries have also been published to provide details on the data science approach and select results of MX in action. This inclusivity not only accelerates the pace of AI advancement but also promotes openness, accountability, and the responsible development of AI applications.
"AMD is pleased to be a founding member of the MX Alliance and has been a key contributor to the OCP MX Specification v1.0. This industry collaboration to standardize MX data formats provides an open and sustainable approach to continued AI innovations while providing the AI ecosystem time to prepare for the use of MX data formats in future hardware and software. AMD is committed to driving forward an open AI ecosystem and is happy to contribute our research results on MX data formats to the broader AI community." - Michael Schulte, Sr. Fellow, AMD
"As an industry we have a unique opportunity to collaborate and realize the benefits of AI technology, which will enable new use cases from cloud to edge to endpoint. This requires commitment to standardization for AI training and inference so that developers can focus on innovating where it really matters, and the release of the OCP MX specification is a significant milestone in this journey." - Ian Bratt, Fellow and Senior Director of Technology, Arm
"The OCP MX spec is the result of a fairly broad cross-industry collaboration and represents an important step forward in unifying and standardizing emerging sub-8bit data formats for AI applications. Portability and interoperability of AI models enabled by this should make AI developers very happy. Benefiting AI applications should see higher levels of performance and energy efficiency, with reduced memory needs." - Pradeep Dubey, Senior Fellow and Director of the Parallel Computing Lab, Intel
"To keep pace with the accelerating demands of AI, innovation must happen across every layer of the stack. The OCP MX effort is a significant leap forward in enabling more scalability and efficiency for the most advanced training and inferencing workloads. MX builds upon years of internal work, and now working together with our valued partners, has evolved into an open standard that will benefit the entire AI ecosystem and industry." - Brian Harry, Technical Fellow, Microsoft
"MX formats with a wide spectrum of sub-8-bit support provide efficient training and inference solutions that can be applied to AI models in various domains, from recommendation models with strict accuracy requirements, to the latest large language models that are latency-sensitive and compute intensive. We believe sharing these MX formats with the OCP and broader ML community will lead to more innovation in AI modeling." - Ajit Mathews, Senior Director of Engineering, Meta AI
"The OCP MX specification is a significant step towards accelerating AI training and inference workloads with sub-8-bit data formats. These formats accelerate applications by reducing memory footprint and bandwidth pressure, also allowing for innovation in math operation implementation. The open format specification enables platform interoperability, benefiting the entire industry." - Paulius Micikevicius, Senior Distinguished Engineer, NVIDIA
"The new OCP MX specification will help accelerate the transition to lower-cost, lower-power server-based forms of AI inference. We are passionate about democratizing AI through lower-cost inference and we are glad to join this effort." - Colin Verrilli, Senior Director, Qualcomm Technologies, Inc
About the Open Compute Project Foundation
The Open Compute Project (OCP) is a collaborative Community of hyperscale data center operators, telecom, colocation providers and enterprise IT users, working with the product and solution vendor ecosystem to develop open innovations deployable from the cloud to the edge. The OCP Foundation is responsible for fostering and serving the OCP Community to meet the market and shape the future, taking hyperscale-led innovations to everyone. Meeting the market is accomplished through addressing challenging market obstacles with open specifications, designs and emerging market programs that showcase OCP-recognized IT equipment and data center facility best practices. Shaping the future includes investing in strategic initiatives and programs that prepare the IT ecosystem for major technology changes, such as AI & ML, optics, advanced cooling techniques, composable memory and silicon. OCP Community-developed open innovations strive to benefit all, optimized through the lens of impact, efficiency, scale and sustainability.
Learn more at: www.opencompute.org.
View at TechPowerUp Main Site
Narrower formats allow silicon to execute more efficient AI calculations per clock cycle, which accelerates model training and inference times. AI models take up less space, which means they require fewer data fetches from memory, and can run with better performance and efficiency. Additionally, fewer bit transfers reduces data movement over the interconnect, which can enhance application performance or cut network costs.
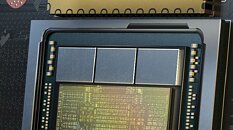
Bringing Together Key Industry Leaders to Set the Standard
Earlier this year, AMD, Arm, Intel, Meta, Microsoft, NVIDIA, and Qualcomm Technologies, Inc. formed the Microscaling Formats (MX) Alliance with the goal of creating and standardizing next-generation 6- and 4-bit data types for AI training and inferencing. The key enabling technology that enables sub 8-bit formats to work, referred to as microscaling, builds on a foundation of years of design space exploration and research. MX enhances the robustness and ease-of-use of existing 8-bit formats such as FP8 and INT8, thus lowering the barrier for broader adoption of single digit bit training and inference.
The initial MX specification introduces four concrete floating point and interger-based data formats (MXFP8, MXFP6, MXFP4, and MXINT8) that are compatible with current AI stacks, upport implementation flexibility across both hardware and software, and enable finegrain microscaling at the hardware level. Extensive studies demonstrate that MX formats can be easily deployed for many diverse real-world cases such as large language models, computer vision, and recommender systems. My technology also enables LLM pre-training at 6- and 4-bit precisions without any modifications to conventional training recipes.
Democratizing AI Capabilities
In the evolving landscape of AI, open standards are critical to foster innovation, collaboration, and widespread adoption. These standards offer a unifying framework that enables consistent toolchains, model development, and interoperability across the AI ecosystem. This further empowers developers and organizations to harness the full potential of AI while mitigating the fragmentation and technology constraints that could otherwise stifle progress.
In this spirit, the MX Alliance has released the Microscaling Formats (MX) Specification v1.0 in an open, license-free format through the Open Compute Project Foundation (OCP) to enable and encourage broad industry adoption and provide the foundation for potential future narrow-format innovations. Additionally, a white paper and emulation libraries have also been published to provide details on the data science approach and select results of MX in action. This inclusivity not only accelerates the pace of AI advancement but also promotes openness, accountability, and the responsible development of AI applications.
"AMD is pleased to be a founding member of the MX Alliance and has been a key contributor to the OCP MX Specification v1.0. This industry collaboration to standardize MX data formats provides an open and sustainable approach to continued AI innovations while providing the AI ecosystem time to prepare for the use of MX data formats in future hardware and software. AMD is committed to driving forward an open AI ecosystem and is happy to contribute our research results on MX data formats to the broader AI community." - Michael Schulte, Sr. Fellow, AMD
"As an industry we have a unique opportunity to collaborate and realize the benefits of AI technology, which will enable new use cases from cloud to edge to endpoint. This requires commitment to standardization for AI training and inference so that developers can focus on innovating where it really matters, and the release of the OCP MX specification is a significant milestone in this journey." - Ian Bratt, Fellow and Senior Director of Technology, Arm
"The OCP MX spec is the result of a fairly broad cross-industry collaboration and represents an important step forward in unifying and standardizing emerging sub-8bit data formats for AI applications. Portability and interoperability of AI models enabled by this should make AI developers very happy. Benefiting AI applications should see higher levels of performance and energy efficiency, with reduced memory needs." - Pradeep Dubey, Senior Fellow and Director of the Parallel Computing Lab, Intel
"To keep pace with the accelerating demands of AI, innovation must happen across every layer of the stack. The OCP MX effort is a significant leap forward in enabling more scalability and efficiency for the most advanced training and inferencing workloads. MX builds upon years of internal work, and now working together with our valued partners, has evolved into an open standard that will benefit the entire AI ecosystem and industry." - Brian Harry, Technical Fellow, Microsoft
"MX formats with a wide spectrum of sub-8-bit support provide efficient training and inference solutions that can be applied to AI models in various domains, from recommendation models with strict accuracy requirements, to the latest large language models that are latency-sensitive and compute intensive. We believe sharing these MX formats with the OCP and broader ML community will lead to more innovation in AI modeling." - Ajit Mathews, Senior Director of Engineering, Meta AI
"The OCP MX specification is a significant step towards accelerating AI training and inference workloads with sub-8-bit data formats. These formats accelerate applications by reducing memory footprint and bandwidth pressure, also allowing for innovation in math operation implementation. The open format specification enables platform interoperability, benefiting the entire industry." - Paulius Micikevicius, Senior Distinguished Engineer, NVIDIA
"The new OCP MX specification will help accelerate the transition to lower-cost, lower-power server-based forms of AI inference. We are passionate about democratizing AI through lower-cost inference and we are glad to join this effort." - Colin Verrilli, Senior Director, Qualcomm Technologies, Inc
About the Open Compute Project Foundation
The Open Compute Project (OCP) is a collaborative Community of hyperscale data center operators, telecom, colocation providers and enterprise IT users, working with the product and solution vendor ecosystem to develop open innovations deployable from the cloud to the edge. The OCP Foundation is responsible for fostering and serving the OCP Community to meet the market and shape the future, taking hyperscale-led innovations to everyone. Meeting the market is accomplished through addressing challenging market obstacles with open specifications, designs and emerging market programs that showcase OCP-recognized IT equipment and data center facility best practices. Shaping the future includes investing in strategic initiatives and programs that prepare the IT ecosystem for major technology changes, such as AI & ML, optics, advanced cooling techniques, composable memory and silicon. OCP Community-developed open innovations strive to benefit all, optimized through the lens of impact, efficiency, scale and sustainability.
Learn more at: www.opencompute.org.
View at TechPowerUp Main Site