T0@st
News Editor
- Joined
- Mar 7, 2023
- Messages
- 2,842 (3.74/day)
- Location
- South East, UK
System Name | The TPU Typewriter |
---|---|
Processor | AMD Ryzen 5 5600 (non-X) |
Motherboard | GIGABYTE B550M DS3H Micro ATX |
Cooling | DeepCool AS500 |
Memory | Kingston Fury Renegade RGB 32 GB (2 x 16 GB) DDR4-3600 CL16 |
Video Card(s) | PowerColor Radeon RX 7800 XT 16 GB Hellhound OC |
Storage | Samsung 980 Pro 1 TB M.2-2280 PCIe 4.0 X4 NVME SSD |
Display(s) | Lenovo Legion Y27q-20 27" QHD IPS monitor |
Case | GameMax Spark M-ATX (re-badged Jonsbo D30) |
Audio Device(s) | FiiO K7 Desktop DAC/Amp + Philips Fidelio X3 headphones, or ARTTI T10 Planar IEMs |
Power Supply | ADATA XPG CORE Reactor 650 W 80+ Gold ATX |
Mouse | Roccat Kone Pro Air |
Keyboard | Cooler Master MasterKeys Pro L |
Software | Windows 10 64-bit Home Edition |
Nexa AI, today, announced NexaQuants of two DeepSeek R1 Distills: The DeepSeek R1 Distill Qwen 1.5B and DeepSeek R1 Distill Llama 8B. Popular quantization methods like the llama.cpp based Q4 K M allow large language models to significantly reduce their memory footprint and typically offer low perplexity loss for dense models as a tradeoff. However, even low perplexity loss can result in a reasoning capability hit for (dense or MoE) models that use Chain of Thought traces. Nexa AI has stated that NexaQuants are able to recover this reasoning capability loss (compared to the full 16-bit precision) while keeping the 4-bit quantization and all the while retaining the performance advantage. Benchmarks provided by Nexa AI can be seen below.
We can see that the Q4 K M quantized DeepSeek R1 distills score slightly less (except for the AIME24 bench on Llama 3 8b distill, which scores significantly lower) in LLM benchmarks like GPQA and AIME24 compared to their full 16-bit counter parts. Moving to a Q6 or Q8 quantization would be one way to fix this problem - but would result in the model becoming slightly slower to run and requiring more memory. Nexa AI has stated that NexaQuants use a proprietary quantization method to recover the loss while keeping the quantization at 4-bits. This means users can theoretically get the best of both worlds: accuracy and speed.
You can read more about the NexaQuant DeepSeek R1 Distills over here.
The following NexaQuants DeepSeek R1 Distills are available for download:
How to run NexaQuants on your AMD Ryzen processors or Radeon graphics card
We recommend using LM Studio for all your LLM needs.
According to this data provided by Nexa AI, developers can also use the NexaQuant versions of the DeepSeek R1 Distills above to get generally improved performance in llama.cpp or GGUF based applications.
View at TechPowerUp Main Site | Source
We can see that the Q4 K M quantized DeepSeek R1 distills score slightly less (except for the AIME24 bench on Llama 3 8b distill, which scores significantly lower) in LLM benchmarks like GPQA and AIME24 compared to their full 16-bit counter parts. Moving to a Q6 or Q8 quantization would be one way to fix this problem - but would result in the model becoming slightly slower to run and requiring more memory. Nexa AI has stated that NexaQuants use a proprietary quantization method to recover the loss while keeping the quantization at 4-bits. This means users can theoretically get the best of both worlds: accuracy and speed.
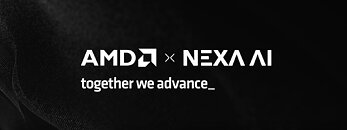
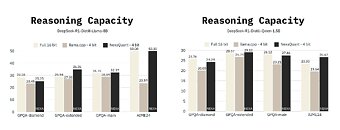
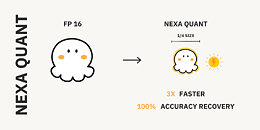
You can read more about the NexaQuant DeepSeek R1 Distills over here.
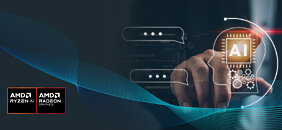
The following NexaQuants DeepSeek R1 Distills are available for download:
How to run NexaQuants on your AMD Ryzen processors or Radeon graphics card
We recommend using LM Studio for all your LLM needs.
- 1) Download and install LM Studio from lmstudio.ai/ryzenai
- 2) Go to the discover tab and paste the huggingface link of one of the nexaquants above.
- 3) Wait for the model to finish downloading.
- 4) Go back to the chat tab and select the model from the drop-down menu. Make sure "manually choose parameters" is selected.
- 5) Set GPU offload layers to MAX.
- 6) Load the model and chat away!
According to this data provided by Nexa AI, developers can also use the NexaQuant versions of the DeepSeek R1 Distills above to get generally improved performance in llama.cpp or GGUF based applications.
View at TechPowerUp Main Site | Source