- Joined
- Aug 19, 2017
- Messages
- 2,971 (1.06/day)
NVIDIA has published a research paper on DLSS version 4, its AI rendering technology for real-time graphics performance. The system integrates advancements in frame generation, ray reconstruction, and latency reduction. The flagship Multi-Frame Generation feature generates three additional frames for every native frame. The DLSS 4 later on brings the best looking frames to the user quickly to make is seem like a real rendering. At the core of DLSS 4 is a shift from convolutional neural networks to transformer models. These new AI architectures excel at capturing spatial-temporal dependencies, improving ray-traced affect quality by 30-50% according to NVIDIA's benchmarks. The technology processes each AI-generated frame in just 1 ms on RTX 5090 GPUs—significantly faster than the 3.25 ms required by DLSS 3. For competitive gaming, the new Reflex Frame Warp feature reduces input latency by up to 75%, achieving 14 ms in THE FINALS and under 3 ms in VALORANT, according to NVIDIA's own benchmarks.
DLSS 4's implementation leverages Blackwell-specific architecture capabilities, including FP8 tensor cores and fused CUDA kernels. The optimized pipeline incorporates vertical layer fusion and memory optimizations that keep computational overhead manageable despite using transformer models, which are twice as large as previous CNN implementations. This efficiency enables real-time performance even with the substantially more complex AI processing. The unified AI pipeline reduces manual tuning requirements for ray-traced effects, allowing studios to implement advanced path tracing across diverse hardware configurations. The design also addresses gaming challenges like interpolating fast-moving UI elements and particle effects and reducing artifacts in high-motion scenes. NVIDIA's hardware flip metering and Blackwell-induced display engine integration ensure precise frame pacing of newly generated frames for smooth, high-refresh-rate gaming, with accurate imagery.
To ensure DLSS works as intended and that the neural networks produce quality results, NVIDIA has used a secret weapon: a dedicated supercomputer that has been continuously improving DLSS for the past six years. The supercomputer's primary task involves analyzing failures in DLSS performance, such as ghosting, flickering, or blurriness across hundreds of games. When issues are identified, the system augments its training data sets with new examples of optimal graphics and challenging scenarios that DLSS needs to address. That way, DLSS learns what games look like and generates realistic frames like a game engine would, without any artifacts.
View at TechPowerUp Main Site | Source
DLSS 4's implementation leverages Blackwell-specific architecture capabilities, including FP8 tensor cores and fused CUDA kernels. The optimized pipeline incorporates vertical layer fusion and memory optimizations that keep computational overhead manageable despite using transformer models, which are twice as large as previous CNN implementations. This efficiency enables real-time performance even with the substantially more complex AI processing. The unified AI pipeline reduces manual tuning requirements for ray-traced effects, allowing studios to implement advanced path tracing across diverse hardware configurations. The design also addresses gaming challenges like interpolating fast-moving UI elements and particle effects and reducing artifacts in high-motion scenes. NVIDIA's hardware flip metering and Blackwell-induced display engine integration ensure precise frame pacing of newly generated frames for smooth, high-refresh-rate gaming, with accurate imagery.
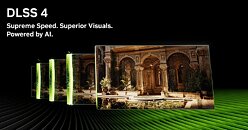
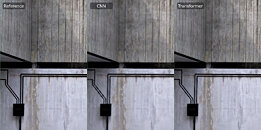
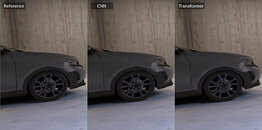
To ensure DLSS works as intended and that the neural networks produce quality results, NVIDIA has used a secret weapon: a dedicated supercomputer that has been continuously improving DLSS for the past six years. The supercomputer's primary task involves analyzing failures in DLSS performance, such as ghosting, flickering, or blurriness across hundreds of games. When issues are identified, the system augments its training data sets with new examples of optimal graphics and challenging scenarios that DLSS needs to address. That way, DLSS learns what games look like and generates realistic frames like a game engine would, without any artifacts.
View at TechPowerUp Main Site | Source