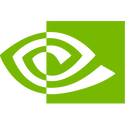
NVIDIA Hopper Leaps Ahead in Generative AI at MLPerf
It's official: NVIDIA delivered the world's fastest platform in industry-standard tests for inference on generative AI. In the latest MLPerf benchmarks, NVIDIA TensorRT-LLM—software that speeds and simplifies the complex job of inference on large language models—boosted the performance of NVIDIA Hopper architecture GPUs on the GPT-J LLM nearly 3x over their results just six months ago. The dramatic speedup demonstrates the power of NVIDIA's full-stack platform of chips, systems and software to handle the demanding requirements of running generative AI. Leading companies are using TensorRT-LLM to optimize their models. And NVIDIA NIM—a set of inference microservices that includes inferencing engines like TensorRT-LLM—makes it easier than ever for businesses to deploy NVIDIA's inference platform.
Raising the Bar in Generative AI
TensorRT-LLM running on NVIDIA H200 Tensor Core GPUs—the latest, memory-enhanced Hopper GPUs—delivered the fastest performance running inference in MLPerf's biggest test of generative AI to date. The new benchmark uses the largest version of Llama 2, a state-of-the-art large language model packing 70 billion parameters. The model is more than 10x larger than the GPT-J LLM first used in the September benchmarks. The memory-enhanced H200 GPUs, in their MLPerf debut, used TensorRT-LLM to produce up to 31,000 tokens/second, a record on MLPerf's Llama 2 benchmark. The H200 GPU results include up to 14% gains from a custom thermal solution. It's one example of innovations beyond standard air cooling that systems builders are applying to their NVIDIA MGX designs to take the performance of Hopper GPUs to new heights.
Raising the Bar in Generative AI
TensorRT-LLM running on NVIDIA H200 Tensor Core GPUs—the latest, memory-enhanced Hopper GPUs—delivered the fastest performance running inference in MLPerf's biggest test of generative AI to date. The new benchmark uses the largest version of Llama 2, a state-of-the-art large language model packing 70 billion parameters. The model is more than 10x larger than the GPT-J LLM first used in the September benchmarks. The memory-enhanced H200 GPUs, in their MLPerf debut, used TensorRT-LLM to produce up to 31,000 tokens/second, a record on MLPerf's Llama 2 benchmark. The H200 GPU results include up to 14% gains from a custom thermal solution. It's one example of innovations beyond standard air cooling that systems builders are applying to their NVIDIA MGX designs to take the performance of Hopper GPUs to new heights.