T0@st
News Editor
- Joined
- Mar 7, 2023
- Messages
- 2,077 (3.18/day)
- Location
- South East, UK
It's official: NVIDIA delivered the world's fastest platform in industry-standard tests for inference on generative AI. In the latest MLPerf benchmarks, NVIDIA TensorRT-LLM—software that speeds and simplifies the complex job of inference on large language models—boosted the performance of NVIDIA Hopper architecture GPUs on the GPT-J LLM nearly 3x over their results just six months ago. The dramatic speedup demonstrates the power of NVIDIA's full-stack platform of chips, systems and software to handle the demanding requirements of running generative AI. Leading companies are using TensorRT-LLM to optimize their models. And NVIDIA NIM—a set of inference microservices that includes inferencing engines like TensorRT-LLM—makes it easier than ever for businesses to deploy NVIDIA's inference platform.
Raising the Bar in Generative AI
TensorRT-LLM running on NVIDIA H200 Tensor Core GPUs—the latest, memory-enhanced Hopper GPUs—delivered the fastest performance running inference in MLPerf's biggest test of generative AI to date. The new benchmark uses the largest version of Llama 2, a state-of-the-art large language model packing 70 billion parameters. The model is more than 10x larger than the GPT-J LLM first used in the September benchmarks. The memory-enhanced H200 GPUs, in their MLPerf debut, used TensorRT-LLM to produce up to 31,000 tokens/second, a record on MLPerf's Llama 2 benchmark. The H200 GPU results include up to 14% gains from a custom thermal solution. It's one example of innovations beyond standard air cooling that systems builders are applying to their NVIDIA MGX designs to take the performance of Hopper GPUs to new heights.
Memory Boost for NVIDIA Hopper GPUs
NVIDIA is shipping H200 GPUs today. They'll be available soon from nearly 20 leading system builders and cloud service providers. H200 GPUs pack 141 GB of HBM3e running at 4.8 TB/s. That's 76% more memory flying 43% faster compared to H100 GPUs. These accelerators plug into the same boards and systems and use the same software as H100 GPUs. With HBM3e memory, a single H200 GPU can run an entire Llama 2 70B model with the highest throughput, simplifying and speeding inference.
GH200 Packs Even More Memory
Even more memory—up to 624 GB of fast memory, including 144 GB of HBM3e—is packed in NVIDIA GH200 Superchips, which combine on one module a Hopper architecture GPU and a power-efficient NVIDIA Grace CPU. NVIDIA accelerators are the first to use HBM3e memory technology. With nearly 5 TB/second memory bandwidth, GH200 Superchips delivered standout performance, including on memory-intensive MLPerf tests such as recommender systems.
Sweeping Every MLPerf Test
On a per-accelerator basis, Hopper GPUs swept every test of AI inference in the latest round of the MLPerf industry benchmarks. The benchmarks cover today's most popular AI workloads and scenarios, including generative AI, recommendation systems, natural language processing, speech and computer vision. NVIDIA was the only company to submit results on every workload in the latest round and every round since MLPerf's data center inference benchmarks began in October 2020.
Continued performance gains translate into lower costs for inference, a large and growing part of the daily work for the millions of NVIDIA GPUs deployed worldwide.
Advancing What's Possible
Pushing the boundaries of what's possible, NVIDIA demonstrated three innovative techniques in a special section of the benchmarks called the open division, created for testing advanced AI methods. NVIDIA engineers used a technique called structured sparsity—a way of reducing calculations, first introduced with NVIDIA A100 Tensor Core GPUs—to deliver up to 33% speedups on inference with Llama 2.
A second open division test found inference speedups of up to 40% using pruning, a way of simplifying an AI model—in this case, an LLM—to increase inference throughput. Finally, an optimization called DeepCache reduced the math required for inference with the Stable Diffusion XL model, accelerating performance by a whopping 74%. All these results were run on NVIDIA H100 Tensor Core GPUs.
A Trusted Source for Users
MLPerf's tests are transparent and objective, so users can rely on the results to make informed buying decisions. NVIDIA's partners participate in MLPerf because they know it's a valuable tool for customers evaluating AI systems and services. Partners submitting results on the NVIDIA AI platform in this round included ASUS, Cisco, Dell Technologies, Fujitsu, GIGABYTE, Google, Hewlett Packard Enterprise, Lenovo, Microsoft Azure, Oracle, QCT, Supermicro, VMware (recently acquired by Broadcom) and Wiwynn.
All the software NVIDIA used in the tests is available in the MLPerf repository. These optimizations are continuously folded into containers available on NGC, NVIDIA's software hub for GPU applications, as well as NVIDIA AI Enterprise—a secure, supported platform that includes NIM inference microservices.
The Next Big Thing
The use cases, model sizes and datasets for generative AI continue to expand. That's why MLPerf continues to evolve, adding real-world tests with popular models like Llama 2 70B and Stable Diffusion XL.
Keeping pace with the explosion in LLM model sizes, NVIDIA founder and CEO Jensen Huang announced last week at GTC that the NVIDIA Blackwell architecture GPUs will deliver new levels of performance required for the multitrillion-parameter AI models.
Inference for large language models is difficult, requiring both expertise and the full-stack architecture NVIDIA demonstrated on MLPerf with Hopper architecture GPUs and TensorRT-LLM. There's much more to come.
Learn more about MLPerf benchmarks and the technical details of this inference round.
View at TechPowerUp Main Site | Source
Raising the Bar in Generative AI
TensorRT-LLM running on NVIDIA H200 Tensor Core GPUs—the latest, memory-enhanced Hopper GPUs—delivered the fastest performance running inference in MLPerf's biggest test of generative AI to date. The new benchmark uses the largest version of Llama 2, a state-of-the-art large language model packing 70 billion parameters. The model is more than 10x larger than the GPT-J LLM first used in the September benchmarks. The memory-enhanced H200 GPUs, in their MLPerf debut, used TensorRT-LLM to produce up to 31,000 tokens/second, a record on MLPerf's Llama 2 benchmark. The H200 GPU results include up to 14% gains from a custom thermal solution. It's one example of innovations beyond standard air cooling that systems builders are applying to their NVIDIA MGX designs to take the performance of Hopper GPUs to new heights.
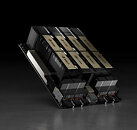
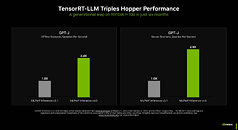
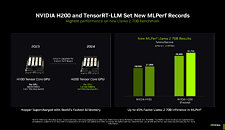
Memory Boost for NVIDIA Hopper GPUs
NVIDIA is shipping H200 GPUs today. They'll be available soon from nearly 20 leading system builders and cloud service providers. H200 GPUs pack 141 GB of HBM3e running at 4.8 TB/s. That's 76% more memory flying 43% faster compared to H100 GPUs. These accelerators plug into the same boards and systems and use the same software as H100 GPUs. With HBM3e memory, a single H200 GPU can run an entire Llama 2 70B model with the highest throughput, simplifying and speeding inference.
GH200 Packs Even More Memory
Even more memory—up to 624 GB of fast memory, including 144 GB of HBM3e—is packed in NVIDIA GH200 Superchips, which combine on one module a Hopper architecture GPU and a power-efficient NVIDIA Grace CPU. NVIDIA accelerators are the first to use HBM3e memory technology. With nearly 5 TB/second memory bandwidth, GH200 Superchips delivered standout performance, including on memory-intensive MLPerf tests such as recommender systems.
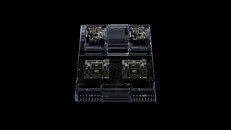
Sweeping Every MLPerf Test
On a per-accelerator basis, Hopper GPUs swept every test of AI inference in the latest round of the MLPerf industry benchmarks. The benchmarks cover today's most popular AI workloads and scenarios, including generative AI, recommendation systems, natural language processing, speech and computer vision. NVIDIA was the only company to submit results on every workload in the latest round and every round since MLPerf's data center inference benchmarks began in October 2020.
Continued performance gains translate into lower costs for inference, a large and growing part of the daily work for the millions of NVIDIA GPUs deployed worldwide.
Advancing What's Possible
Pushing the boundaries of what's possible, NVIDIA demonstrated three innovative techniques in a special section of the benchmarks called the open division, created for testing advanced AI methods. NVIDIA engineers used a technique called structured sparsity—a way of reducing calculations, first introduced with NVIDIA A100 Tensor Core GPUs—to deliver up to 33% speedups on inference with Llama 2.
A second open division test found inference speedups of up to 40% using pruning, a way of simplifying an AI model—in this case, an LLM—to increase inference throughput. Finally, an optimization called DeepCache reduced the math required for inference with the Stable Diffusion XL model, accelerating performance by a whopping 74%. All these results were run on NVIDIA H100 Tensor Core GPUs.
A Trusted Source for Users
MLPerf's tests are transparent and objective, so users can rely on the results to make informed buying decisions. NVIDIA's partners participate in MLPerf because they know it's a valuable tool for customers evaluating AI systems and services. Partners submitting results on the NVIDIA AI platform in this round included ASUS, Cisco, Dell Technologies, Fujitsu, GIGABYTE, Google, Hewlett Packard Enterprise, Lenovo, Microsoft Azure, Oracle, QCT, Supermicro, VMware (recently acquired by Broadcom) and Wiwynn.
All the software NVIDIA used in the tests is available in the MLPerf repository. These optimizations are continuously folded into containers available on NGC, NVIDIA's software hub for GPU applications, as well as NVIDIA AI Enterprise—a secure, supported platform that includes NIM inference microservices.
The Next Big Thing
The use cases, model sizes and datasets for generative AI continue to expand. That's why MLPerf continues to evolve, adding real-world tests with popular models like Llama 2 70B and Stable Diffusion XL.
Keeping pace with the explosion in LLM model sizes, NVIDIA founder and CEO Jensen Huang announced last week at GTC that the NVIDIA Blackwell architecture GPUs will deliver new levels of performance required for the multitrillion-parameter AI models.
Inference for large language models is difficult, requiring both expertise and the full-stack architecture NVIDIA demonstrated on MLPerf with Hopper architecture GPUs and TensorRT-LLM. There's much more to come.
Learn more about MLPerf benchmarks and the technical details of this inference round.
View at TechPowerUp Main Site | Source