- Joined
- May 21, 2024
- Messages
- 1,144 (3.47/day)
Google has rolled out its seventh-generation AI chip, Ironwood, which aims to boost AI application performance. This processor focuses on "inference" computing—the quick calculations needed for chatbot answers and other AI outputs. Ironwood stands as one of the few real options to NVIDIA's leading AI processors coming from Google's ten-year multi-billion-dollar push to develop it. These tensor processing units (TPUs) are exclusively available through Google's cloud service or to its internal engineers.
According to Google Vice President Amin Vahdat, Ironwood combines functions from previously separate designs while increasing memory capacity. The chip can operate in groups of up to 9,216 processors and delivers twice the performance-per-energy ratio compared to last year's Trillium chip. When configured in pods of 9,216 chips, Ironwood delivers 42.5 Exaflops of computing power. This is more than 24 times the computational capacity of El Capitan, currently the world's largest supercomputer, which provides only 1.7 Exaflops per pod.
Ironwood's key features
Google uses these proprietary chips to build and deploy its Gemini AI models. The manufacturer producing the Google-designed processors remains undisclosed.
View at TechPowerUp Main Site | Source
According to Google Vice President Amin Vahdat, Ironwood combines functions from previously separate designs while increasing memory capacity. The chip can operate in groups of up to 9,216 processors and delivers twice the performance-per-energy ratio compared to last year's Trillium chip. When configured in pods of 9,216 chips, Ironwood delivers 42.5 Exaflops of computing power. This is more than 24 times the computational capacity of El Capitan, currently the world's largest supercomputer, which provides only 1.7 Exaflops per pod.
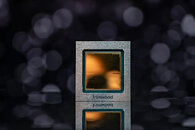
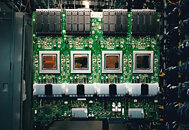
Ironwood's key features
- Ironwood perf/watt is 2x relative to Trillium, Google sixth generation tensor processing units (TPU) announced last year
- Offers 192 GB per chip, 6x that of Trillium
- Improved HBM bandwidth, reaching 7.2 Tbps per chip, 4.5x of Trillium's
- Enhanced Inter-Chip Interconnect (ICI) bandwidth, increased to 1.2 Tbps bidirectional, 1.5x of Trillium's
Google uses these proprietary chips to build and deploy its Gemini AI models. The manufacturer producing the Google-designed processors remains undisclosed.
View at TechPowerUp Main Site | Source