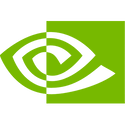
NVIDIA Blackwell Sets New Standard for Generative AI in MLPerf Inference Benchmark
As enterprises race to adopt generative AI and bring new services to market, the demands on data center infrastructure have never been greater. Training large language models is one challenge, but delivering LLM-powered real-time services is another. In the latest round of MLPerf industry benchmarks, Inference v4.1, NVIDIA platforms delivered leading performance across all data center tests. The first-ever submission of the upcoming NVIDIA Blackwell platform revealed up to 4x more performance than the NVIDIA H100 Tensor Core GPU on MLPerf's biggest LLM workload, Llama 2 70B, thanks to its use of a second-generation Transformer Engine and FP4 Tensor Cores.
The NVIDIA H200 Tensor Core GPU delivered outstanding results on every benchmark in the data center category - including the latest addition to the benchmark, the Mixtral 8x7B mixture of experts (MoE) LLM, which features a total of 46.7 billion parameters, with 12.9 billion parameters active per token. MoE models have gained popularity as a way to bring more versatility to LLM deployments, as they're capable of answering a wide variety of questions and performing more diverse tasks in a single deployment. They're also more efficient since they only activate a few experts per inference - meaning they deliver results much faster than dense models of a similar size.
The NVIDIA H200 Tensor Core GPU delivered outstanding results on every benchmark in the data center category - including the latest addition to the benchmark, the Mixtral 8x7B mixture of experts (MoE) LLM, which features a total of 46.7 billion parameters, with 12.9 billion parameters active per token. MoE models have gained popularity as a way to bring more versatility to LLM deployments, as they're capable of answering a wide variety of questions and performing more diverse tasks in a single deployment. They're also more efficient since they only activate a few experts per inference - meaning they deliver results much faster than dense models of a similar size.