Wednesday, March 4th 2020
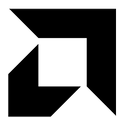
AMD Scores Another EPYC Win in Exascale Computing With DOE's "El Capitan" Two-Exaflop Supercomputer
AMD has been on a roll in both consumer, professional, and exascale computing environments, and it has just snagged itself another hugely important contract. The US Department of Energy (DOE) has just announced the winners for their next-gen, exascale supercomputer that aims to be the world's fastest. Dubbed "El Capitan", the new supercomputer will be powered by AMD's next-gen EPYC Genoa processors (Zen 4 architecture) and Radeon GPUs. This is the first such exascale contract where AMD is the sole purveyor of both CPUs and GPUs, with AMD's other design win with EPYC in the Cray Shasta being paired with NVIDIA graphics cards.
El Capitan will be a $600 million investment to be deployed in late 2022 and operational in 2023. Undoubtedly, next-gen proposals from AMD, Intel and NVIDIA were presented, with AMD winning the shootout in a big way. While initially the DOE projected El Capitan to provide some 1.5 exaflops of computing power, it has now revised their performance goals to a pure 2 exaflop machine. El Capitan willl thus be ten times faster than the current leader of the supercomputing world, Summit.AMD's ability to provide an ecosystem with both CPUs and GPUs very likely played a key part in the DOE's choice for the project, and this all but guarantees that the contractor was left very satisfied with AMD's performance projections for both their Zen 4 and future GPU architectures. AMD's EPYC Genoa will feature support next-gen memory, implying DDR5 or later, and also feature unspecified next-gen I/O connections. AMD's graphics cards aren't detailed at all - they're just referred to as being part of the Radeon instinct lineup featuring a "new compute architecture".
Another wholly important part of this design win has to be that AMD has redesigned their 3rd Gen Infinity Fabric (which supports a 4:1 ratio of GPUs to CPUs) to provide data coherence between CPU and GPU - thus effectively reducing the need for data to move back and forth between the CPU and GPU as it is being processed. With relevant data being mirrored across both pieces of hardware through their coherent, Infinity Fabric-powered memory, computing efficiency can be significantly improved (since data transition usually requires more power expenditure than the actual computing calculations themselves), and that too must've played a key part in the selection.El Capitan will also feature a future version of CRAY's proprietary Slingshot network fabric for increased speed and reduced latencies. All of this will be tied together with AMD's ROCm open software platform for heterogeneous programming to maximize performance of the CPUs and GPUs in OpenMP environments. ROCm has recently gotten a pretty healthy, $100 million shot in the arm also courtesy of the DOE, having deployed a Center of Excellence at the Lawrence Livermore National Lab (part of the DOE) to help develop ROCm. So this means AMD's software arm too is flexing its muscles - for this kind of deployment, at least - which has always been a contention point against rival NVIDIA, who has typically shown to invest much more in its software implementations than AMD - and hence the reason NVIDIA has been such a big player in the enterprise and computing segments until now.As for why NVIDIA was shunned, it likely has nothing to do with their next-gen designs offering lesser performance than what AMD brought to the table. If anything, I'd take an educated guess in that the 3rd gen Infinity Fabric and its memory coherence was the deciding factor in choosing AMD GPUs over NVIDIA's, because the green company doesn't have anything like that to offer - it doesn't play in the x64 computing space, and can't offer that level of platform interconnectedness. Whatever the reason, this is yet another big win for AMD, who keeps muscling Intel out of very, very lucrative positions.
Source:
Tom's Hardware
El Capitan will be a $600 million investment to be deployed in late 2022 and operational in 2023. Undoubtedly, next-gen proposals from AMD, Intel and NVIDIA were presented, with AMD winning the shootout in a big way. While initially the DOE projected El Capitan to provide some 1.5 exaflops of computing power, it has now revised their performance goals to a pure 2 exaflop machine. El Capitan willl thus be ten times faster than the current leader of the supercomputing world, Summit.AMD's ability to provide an ecosystem with both CPUs and GPUs very likely played a key part in the DOE's choice for the project, and this all but guarantees that the contractor was left very satisfied with AMD's performance projections for both their Zen 4 and future GPU architectures. AMD's EPYC Genoa will feature support next-gen memory, implying DDR5 or later, and also feature unspecified next-gen I/O connections. AMD's graphics cards aren't detailed at all - they're just referred to as being part of the Radeon instinct lineup featuring a "new compute architecture".
Another wholly important part of this design win has to be that AMD has redesigned their 3rd Gen Infinity Fabric (which supports a 4:1 ratio of GPUs to CPUs) to provide data coherence between CPU and GPU - thus effectively reducing the need for data to move back and forth between the CPU and GPU as it is being processed. With relevant data being mirrored across both pieces of hardware through their coherent, Infinity Fabric-powered memory, computing efficiency can be significantly improved (since data transition usually requires more power expenditure than the actual computing calculations themselves), and that too must've played a key part in the selection.El Capitan will also feature a future version of CRAY's proprietary Slingshot network fabric for increased speed and reduced latencies. All of this will be tied together with AMD's ROCm open software platform for heterogeneous programming to maximize performance of the CPUs and GPUs in OpenMP environments. ROCm has recently gotten a pretty healthy, $100 million shot in the arm also courtesy of the DOE, having deployed a Center of Excellence at the Lawrence Livermore National Lab (part of the DOE) to help develop ROCm. So this means AMD's software arm too is flexing its muscles - for this kind of deployment, at least - which has always been a contention point against rival NVIDIA, who has typically shown to invest much more in its software implementations than AMD - and hence the reason NVIDIA has been such a big player in the enterprise and computing segments until now.As for why NVIDIA was shunned, it likely has nothing to do with their next-gen designs offering lesser performance than what AMD brought to the table. If anything, I'd take an educated guess in that the 3rd gen Infinity Fabric and its memory coherence was the deciding factor in choosing AMD GPUs over NVIDIA's, because the green company doesn't have anything like that to offer - it doesn't play in the x64 computing space, and can't offer that level of platform interconnectedness. Whatever the reason, this is yet another big win for AMD, who keeps muscling Intel out of very, very lucrative positions.
35 Comments on AMD Scores Another EPYC Win in Exascale Computing With DOE's "El Capitan" Two-Exaflop Supercomputer
I guess whatever DOE uses probably does not rely on CUDA or Tensorflow.
Unless one have massive amount of resource to invest in building something from ground up in OpenCL, CUDA is THE best option for accelerated computing.
TBH I prefer Vulkan compute over OpenCL. Better in every single way
Guess CUDA and a locked in eco-system isn't all that afterall.
Also likely means AMD is bringing AI processing to the next gen stuff.
Just because universities still use Apple's in their media programs doesn't mean they are the best. It's just what they've used and still do. Just saying...
Also shouldn't Vulkan's GP-GPU stuff be better than OpenCL since it replaced OpenGL basically. OpenCL is ancient by this point.
If anything, those same corporations would employ researchers from these universities. :laugh: Why do you keep saying CUDA is in a locked-in eco-system? You can run CUDA code on other hardware (even on x86 and ARM, if you're desperate) using HIP through ROCm, but you need to translate (not manual conversion) to avoid any NVIDIA extensions. This is currently a lot more efficient than what can be done in OpenCL 2.1.
The investment in ROCm is an advantage for everyone since all compute APIs will use this. Thank AMD for pulling this off. They still use Apple because of deals (think 60%+ hardware and support discounts) offered by Apple. Also hardware deployment of Mac minis and Pros depends on department use cases.
Vulkan is aimed at rendering (and why any GPGPU code using Vulkan is on the graphics pipeline), which is why it succeeds OpenGL. OpenCL is meant for GPGPU use.
averaging >5kw of power per u of compute space... 16,000 cpus and 64,000 gpus... :D
The Fast Forward 2 project is heterogeneous. One mistake, we thought it would be comprised of apus whereas epyc+RI with heterogeneous(coherent) memory is the underlying hardware.
Superior technology speaks louder than anything, which is also the reason all of our new nodes added are EPYC2 based.
And nvidia doesn't like custom... they want you to use dgx reference boards. This is far denser than anything that could be achieved any other way.
While ROCm is still not at cuda parity its getting there... And their hip c++ compiler is pretty baller, you can take cuda code, compile it to C with AMDs tool and it run faster than native cuda on an nvidia card...
Also... While ROCm's support lags a version behind on things like tensorflow may be annoying and unacceptable elsewhere...it's faster than any government code is pushed out...
The main reason why CUDA ruled the HPC and GPGPU compute in general, is being fast. Other aspects are just a consequence of the first one.