Monday, September 11th 2023
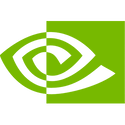
NVIDIA GH200 Superchip Aces MLPerf Inference Benchmarks
In its debut on the MLPerf industry benchmarks, the NVIDIA GH200 Grace Hopper Superchip ran all data center inference tests, extending the leading performance of NVIDIA H100 Tensor Core GPUs. The overall results showed the exceptional performance and versatility of the NVIDIA AI platform from the cloud to the network's edge. Separately, NVIDIA announced inference software that will give users leaps in performance, energy efficiency and total cost of ownership.
GH200 Superchips Shine in MLPerf
The GH200 links a Hopper GPU with a Grace CPU in one superchip. The combination provides more memory, bandwidth and the ability to automatically shift power between the CPU and GPU to optimize performance. Separately, NVIDIA HGX H100 systems that pack eight H100 GPUs delivered the highest throughput on every MLPerf Inference test in this round. Grace Hopper Superchips and H100 GPUs led across all MLPerf's data center tests, including inference for computer vision, speech recognition and medical imaging, in addition to the more demanding use cases of recommendation systems and the large language models (LLMs) used in generative AI.Overall, the results continue NVIDIA's record of demonstrating performance leadership in AI training and inference in every round since the launch of the MLPerf benchmarks in 2018. The latest MLPerf round included an updated test of recommendation systems, as well as the first inference benchmark on GPT-J, an LLM with six billion parameters, a rough measure of an AI model's size.
TensorRT-LLM Supercharges Inference
To cut through complex workloads of every size, NVIDIA developed TensorRT-LLM, generative AI software that optimizes inference. The open-source library — which was not ready in time for August submission to MLPerf—enables customers to more than double the inference performance of their already purchased H100 GPUs at no added cost.NVIDIA's internal tests show that using TensorRT-LLM on H100 GPUs provides up to an 8x performance speedup compared to prior generation GPUs running GPT-J 6B without the software.
The software got its start in NVIDIA's work accelerating and optimizing LLM inference with leading companies including Meta, AnyScale, Cohere, Deci, Grammarly, Mistral AI, MosaicML (now part of Databricks), OctoML, Tabnine and Together AI.
MosaicML added features that it needs on top of TensorRT-LLM and integrated them into its existing serving stack. "It's been an absolute breeze," said Naveen Rao, vice president of engineering at Databricks.
"TensorRT-LLM is easy-to-use, feature-packed and efficient," Rao said. "It delivers state-of-the-art performance for LLM serving using NVIDIA GPUs and allows us to pass on the cost savings to our customers."
TensorRT-LLM is the latest example of continuous innovation on NVIDIA's full-stack AI platform. These ongoing software advances give users performance that grows over time at no extra cost and is versatile across diverse AI workloads.
L4 Boosts Inference on Mainstream Servers
In the latest MLPerf benchmarks, NVIDIA L4 GPUs ran the full range of workloads and delivered great performance across the board. For example, L4 GPUs running in compact, 72 W PCIe accelerators delivered up to 6x more performance than CPUs rated for nearly 5x higher power consumption. In addition, L4 GPUs feature dedicated media engines that, in combination with CUDA software, provide up to 120x speedups for computer vision in NVIDIA's tests.
L4 GPUs are available from Google Cloud and many system builders, serving customers in industries from consumer internet services to drug discovery.
Performance Boosts at the Edge
Separately, NVIDIA applied a new model compression technology to demonstrate up to a 4.7x performance boost running the BERT LLM on an L4 GPU. The result was in MLPerf's so-called "open division," a category for showcasing new capabilities. The technique is expected to find use across all AI workloads. It can be especially valuable when running models on edge devices constrained by size and power consumption.
In another example of leadership in edge computing, the NVIDIA Jetson Orin system-on-module showed performance increases of up to 84% compared to the prior round in object detection, a computer vision use case common in edge AI and robotics scenarios.The Jetson Orin advance came from software taking advantage of the latest version of the chip's cores, such as a programmable vision accelerator, an NVIDIA Ampere architecture GPU and a dedicated deep learning accelerator.
Versatile Performance, Broad Ecosystem
The MLPerf benchmarks are transparent and objective, so users can rely on their results to make informed buying decisions. They also cover a wide range of use cases and scenarios, so users know they can get performance that's both dependable and flexible to deploy. Partners submitting in this round included cloud service providers Microsoft Azure and Oracle Cloud Infrastructure and system manufacturers ASUS, Connect Tech, Dell Technologies, Fujitsu, GIGABYTE, Hewlett Packard Enterprise, Lenovo, QCT and Supermicro.
Overall, MLPerf is backed by more than 70 organizations, including Alibaba, Arm, Cisco, Google, Harvard University, Intel, Meta, Microsoft and the University of Toronto.
Read a technical blog for more details on how NVIDIA achieved the latest results.
All the software used in NVIDIA's benchmarks is available from the MLPerf repository, so everyone can get the same world-class results. The optimizations are continuously folded into containers available on the NVIDIA NGC software hub for GPU applications.
Source:
NVIDIA News
GH200 Superchips Shine in MLPerf
The GH200 links a Hopper GPU with a Grace CPU in one superchip. The combination provides more memory, bandwidth and the ability to automatically shift power between the CPU and GPU to optimize performance. Separately, NVIDIA HGX H100 systems that pack eight H100 GPUs delivered the highest throughput on every MLPerf Inference test in this round. Grace Hopper Superchips and H100 GPUs led across all MLPerf's data center tests, including inference for computer vision, speech recognition and medical imaging, in addition to the more demanding use cases of recommendation systems and the large language models (LLMs) used in generative AI.Overall, the results continue NVIDIA's record of demonstrating performance leadership in AI training and inference in every round since the launch of the MLPerf benchmarks in 2018. The latest MLPerf round included an updated test of recommendation systems, as well as the first inference benchmark on GPT-J, an LLM with six billion parameters, a rough measure of an AI model's size.
TensorRT-LLM Supercharges Inference
To cut through complex workloads of every size, NVIDIA developed TensorRT-LLM, generative AI software that optimizes inference. The open-source library — which was not ready in time for August submission to MLPerf—enables customers to more than double the inference performance of their already purchased H100 GPUs at no added cost.NVIDIA's internal tests show that using TensorRT-LLM on H100 GPUs provides up to an 8x performance speedup compared to prior generation GPUs running GPT-J 6B without the software.
The software got its start in NVIDIA's work accelerating and optimizing LLM inference with leading companies including Meta, AnyScale, Cohere, Deci, Grammarly, Mistral AI, MosaicML (now part of Databricks), OctoML, Tabnine and Together AI.
MosaicML added features that it needs on top of TensorRT-LLM and integrated them into its existing serving stack. "It's been an absolute breeze," said Naveen Rao, vice president of engineering at Databricks.
"TensorRT-LLM is easy-to-use, feature-packed and efficient," Rao said. "It delivers state-of-the-art performance for LLM serving using NVIDIA GPUs and allows us to pass on the cost savings to our customers."
TensorRT-LLM is the latest example of continuous innovation on NVIDIA's full-stack AI platform. These ongoing software advances give users performance that grows over time at no extra cost and is versatile across diverse AI workloads.
L4 Boosts Inference on Mainstream Servers
In the latest MLPerf benchmarks, NVIDIA L4 GPUs ran the full range of workloads and delivered great performance across the board. For example, L4 GPUs running in compact, 72 W PCIe accelerators delivered up to 6x more performance than CPUs rated for nearly 5x higher power consumption. In addition, L4 GPUs feature dedicated media engines that, in combination with CUDA software, provide up to 120x speedups for computer vision in NVIDIA's tests.
L4 GPUs are available from Google Cloud and many system builders, serving customers in industries from consumer internet services to drug discovery.
Performance Boosts at the Edge
Separately, NVIDIA applied a new model compression technology to demonstrate up to a 4.7x performance boost running the BERT LLM on an L4 GPU. The result was in MLPerf's so-called "open division," a category for showcasing new capabilities. The technique is expected to find use across all AI workloads. It can be especially valuable when running models on edge devices constrained by size and power consumption.
In another example of leadership in edge computing, the NVIDIA Jetson Orin system-on-module showed performance increases of up to 84% compared to the prior round in object detection, a computer vision use case common in edge AI and robotics scenarios.The Jetson Orin advance came from software taking advantage of the latest version of the chip's cores, such as a programmable vision accelerator, an NVIDIA Ampere architecture GPU and a dedicated deep learning accelerator.
Versatile Performance, Broad Ecosystem
The MLPerf benchmarks are transparent and objective, so users can rely on their results to make informed buying decisions. They also cover a wide range of use cases and scenarios, so users know they can get performance that's both dependable and flexible to deploy. Partners submitting in this round included cloud service providers Microsoft Azure and Oracle Cloud Infrastructure and system manufacturers ASUS, Connect Tech, Dell Technologies, Fujitsu, GIGABYTE, Hewlett Packard Enterprise, Lenovo, QCT and Supermicro.
Overall, MLPerf is backed by more than 70 organizations, including Alibaba, Arm, Cisco, Google, Harvard University, Intel, Meta, Microsoft and the University of Toronto.
Read a technical blog for more details on how NVIDIA achieved the latest results.
All the software used in NVIDIA's benchmarks is available from the MLPerf repository, so everyone can get the same world-class results. The optimizations are continuously folded into containers available on the NVIDIA NGC software hub for GPU applications.
Comments on NVIDIA GH200 Superchip Aces MLPerf Inference Benchmarks
There are no comments yet.