Monday, February 20th 2023
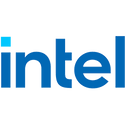
Intel Publishes Sorting Library Powered by AVX-512, Offers 10-17x Speed Up
Intel has recently updated its open-source C++ header file library for high-performance SIMD-based sorting to support the AVX-512 SIMD instruction set. Extending the capability of regular AVX2 support, the sorting functions now implement 512-bit extensions to offer greater performance. According to Phoronix, the NumPy Python library for mathematics that underpins a lot of software has updated its software base to use the AVX-512 boosted sorting functionality that yields a fantastic uplift in performance. The library uses AVX-512 to vectorize the quicksort for 16-bit and 64-bit data types using the extended instruction set. Benchmarked on an Intel Tiger Lake system, the NumPy sorting saw a 10-17x increase in performance.
Intel's engineer Raghuveer Devulapalli changed the NumPy code, which was merged into the NumPy codebase on Wednesday. Regarding individual data types, the new implementation increases 16-bit int sorting by 17x and 32-bit data type sorting by 12-13x, while float 64-bit sorting for random arrays has experienced a 10x speed up. Using the x86-simd-sort code, this speed-up shows the power of AVX-512 and its capability to enhance the performance of various libraries. We hope to see more implementations of AVX-512, as AMD has joined the party by placing AVX-512 processing elements on Zen 4.
Source:
Phoronix
Intel's engineer Raghuveer Devulapalli changed the NumPy code, which was merged into the NumPy codebase on Wednesday. Regarding individual data types, the new implementation increases 16-bit int sorting by 17x and 32-bit data type sorting by 12-13x, while float 64-bit sorting for random arrays has experienced a 10x speed up. Using the x86-simd-sort code, this speed-up shows the power of AVX-512 and its capability to enhance the performance of various libraries. We hope to see more implementations of AVX-512, as AMD has joined the party by placing AVX-512 processing elements on Zen 4.
28 Comments on Intel Publishes Sorting Library Powered by AVX-512, Offers 10-17x Speed Up
I'm still not sold on AVX-512 being a necessity to consumer segment chips, AMD fans have long pointed out to it being worthless... until Zen 4 supported it anyway and then they forgot they held Linus Torvalds in the esteem of a God for saying that it should die.
Knowing Intel I bet this is set up such that it chooses non AVX-512 code path for anything that isn't Intel, so this is going to run on... nothing that is current gen I guess ? Lol.
Also AMDs support apparently isnt entirely the same as what intel is doing
So yes, it was a smart move by AMD.
By example, no games would spend time right now trying to find way to make their game run faster for just the few folks that have TigerLake and Zen 4 CPU. (or the very few that run Alder Lake without the E-Core to get AVX-512 before intel disabled it).
But that will come. We don't know how useful it will be until it start being utilized.
As for instruction support, it's a hot mess. But mostly a hot mess on Intel side.
en.wikipedia.org/wiki/AVX-512#CPUs_with_AVX-512 They will probably add it again in future generation when their E core (if they continue on that path) will have it. The Intel implementation of AVX512 take a lot of silicon area. This is against the goal of E-core to be really small in die size.
One approach to emulate AVX-512 instructions is to use a combination of SSE and AVX2 instructions. This approach can achieve similar results to AVX-512 but with reduced performance. The following code snippet shows an example of how to emulate the 512-bit vector addition operation using SSE and AVX2 instructions:
cCopy code
#include <immintrin.h> void add_avx512_emulation(float *a, float *b, float *c, int n) {int i; __m256 v0, v1, v2, v3; __m128 w0, w1, w2, w3; for (i = 0; i < n; i += 16) { v0 = _mm256_load_ps(&a); v1 = _mm256_load_ps(&a[i + 8]); v2 = _mm256_load_ps(&b); v3 = _mm256_load_ps(&b[i + 8]); w0 = _mm_add_ps(_mm256_castps256_ps128(v0), _mm256_castps256_ps128(v2)); w1 = _mm_add_ps(_mm256_extractf128_ps(v0, 1), _mm256_extractf128_ps(v2, 1)); w2 = _mm_add_ps(_mm256_castps256_ps128(v1), _mm256_castps256_ps128(v3)); w3 = _mm_add_ps(_mm256_extractf128_ps(v1, 1), _mm256_extractf128_ps(v3, 1)); v0 = _mm256_castps128_ps256(w0); v1 = _mm256_insertf128_ps(v1, w1, 1); v2 = _mm256_castps128_ps256(w2); v3 = _mm256_insertf128_ps(v3, w3, 1); _mm256_store_ps(&c, _mm256_add_ps(v0, v2)); _mm256_store_ps(&c[i + 8], _mm256_add_ps(v1, v3)); } }
In this example, the 512-bit vectors are decomposed into four 128-bit vectors and then operated on in two steps. First, the 128-bit vectors are added using SSE instructions. Then, the resulting 128-bit vectors are combined to form the final 512-bit vectors using AVX2 instructions. This emulation technique can be extended to other AVX-512 instructions as well.
Note that emulating AVX-512 instructions using a combination of SSE and AVX2 instructions can be useful in scenarios where AVX-512 support is not available.
To increase the performance of an emulation technique that uses a combination of SSE and AVX2 instructions to emulate AVX-512, you can try the following techniques:
- Loop unrolling: Unrolling the loop can improve the performance by reducing the number of iterations and increasing the arithmetic intensity of the loop body. For example, you can unroll the loop by a factor of two or four, depending on the available registers and the data dependencies in the loop body.
- Memory alignment: Memory alignment can significantly improve the performance by reducing the number of memory accesses and improving the cache locality. Ensure that the data is aligned to the cache line size and that the load and store operations use aligned memory addresses.
- Code optimization: Code optimization can improve the performance by reducing the number of instructions and improving the instruction pipeline efficiency. Techniques such as loop-invariant code motion, common subexpression elimination, and dead-code elimination can reduce the number of instructions and improve the instruction pipeline efficiency.
- Processor-specific optimizations: Processor-specific optimizations can improve the performance by taking advantage of the specific features of the processor. For example, some processors have specialized instructions that can improve the performance of specific operations. By using these instructions, you can improve the performance of your emulation technique.
- Data format optimization: Data format optimization can improve the performance by using data formats that are optimized for the specific operations. For example, using a packed data format can reduce the number of instructions and improve the performance of vector operations.
Note that the above techniques can improve the performance of an emulation technique, but they may not be able to match the performance of the native AVX-512 instructions.Users can -and should- manually set which feature level to target (or enable native optimizations, which checks what CPU support and enables features accordingly). Which is quite common, afaik. Even your own code would throw errors if you used AVX2/512 functions without setting appropriate flags in your compiler.
Most AVX512 subfeatures(?) are grouped into levels conforming to Intel's SKU generations, but that's mostly due to the fact that AMD wasn't offering any support for them. Skimming over the commit, I spotted a comment about a planned AVX512_ZEN4 grouping, so it's just a matter of time.
consumer: avx512 or addition core? (Or e cores)
avx512 is very powerful for edge case workloads
x consumer
Alder processors with the old Intel logo have full support, like Xeon.
No, I don't miss them.