Thursday, May 21st 2015
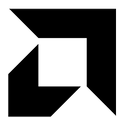
Tech Source Releases Condor 4000 3U VPX Graphics Card for GPGPU Applications
Tech Source, Inc., an independent supplier of high performance embedded video, graphics, and high end computing solutions, has released the Condor 4000 3U VPX form factor graphics/video card. Designed for compute-intensive General Purpose Graphics Processing Unit (GPGPU) applications deployed in avionics and military technology, the Condor 4000 3U VPX graphics card delivers up to 768/48 GFLOPS of peak single/double precision floating point performance and 640 shaders with the AMD Radeon E8860 GPU at its core.
The Condor 4000 3U VPX card is for seriously high-end graphics, parallel processing, and situation awareness image and sensor processing applications such as radar, sonar, video streaming, and unmanned systems. The new card operates at higher speeds than an XMC form factor equivalent card as it occupies a dedicated slot to allow for better cooling which enables it to run at 45 Watts full power.Selwyn L. Henriques, president and CEO of Tech Source Inc., commented, "Our GPGPU customers want every ounce of performance they can get. So this 3U VPX graphics card is an attractive option as it delivers 60 percent better performance than the previous generation."
The Condor 4000 3U VPX board is fully conduction-cooled and has 6 digital video outputs (2 x DVI and 4 x DisplayPort) available from the rear VPX P2 connector on the card. It also features 2 GB of GDDR5 memory and supports the latest versions of the APIs such as Open GL 4.2, Direct X 11.1, OpenCL 1.2, and DirectCompute 11 for GPGPU computing.
The Condor 4000 3U VPX card is available with Linux and Windows drivers by default and other real time operating systems such as VxWorks may be supported. Tech Source offers 15 years product support and a board customizing service for those with specialized requirements.
The Condor 4000 3U VPX card is for seriously high-end graphics, parallel processing, and situation awareness image and sensor processing applications such as radar, sonar, video streaming, and unmanned systems. The new card operates at higher speeds than an XMC form factor equivalent card as it occupies a dedicated slot to allow for better cooling which enables it to run at 45 Watts full power.Selwyn L. Henriques, president and CEO of Tech Source Inc., commented, "Our GPGPU customers want every ounce of performance they can get. So this 3U VPX graphics card is an attractive option as it delivers 60 percent better performance than the previous generation."
The Condor 4000 3U VPX board is fully conduction-cooled and has 6 digital video outputs (2 x DVI and 4 x DisplayPort) available from the rear VPX P2 connector on the card. It also features 2 GB of GDDR5 memory and supports the latest versions of the APIs such as Open GL 4.2, Direct X 11.1, OpenCL 1.2, and DirectCompute 11 for GPGPU computing.
The Condor 4000 3U VPX card is available with Linux and Windows drivers by default and other real time operating systems such as VxWorks may be supported. Tech Source offers 15 years product support and a board customizing service for those with specialized requirements.
8 Comments on Tech Source Releases Condor 4000 3U VPX Graphics Card for GPGPU Applications
Lets say we wanted to map the oceans, how do you determine where you are when GPS doesn't work under water? Get a fixed location, use a combination of sensors to pick a few points to triangulate a location (same way we have a camera angle in games to determine distance to an item) then use them as reference points, and the ability to use a programmable board with things like varying filters based on expected feedback VS actual feedback is new, before we had hardware filters built in and they may have been able to work in perfectly clear water, but add in debris and it became inaccurate, or thermal convection causes scintillation and distortion, so being able to run a kalman filter on multiple systems and deterministically choose the highest accuracy one is new and a huge improvement.
I was just curious if in this specific application they were running into that problem with hardware based Kalman filters. If that makes sense.
Only experience I have is with mining using FPGA's, and the software was pre written but could be easily modified.