Friday, January 17th 2025
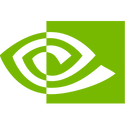
NVIDIA Reveals Secret Weapon Behind DLSS Evolution: Dedicated Supercomputer Running for Six Years
At the RTX "Blackwell" Editor's Day during CES 2025, NVIDIA pulled back the curtain on one of its most powerful tools: a dedicated supercomputer that has been continuously improving DLSS (Deep Learning Super Sampling) for the past six years. Brian Catanzaro, NVIDIA's VP of applied deep learning research, disclosed that thousands of the company's latest GPUs have been working round-the-clock, analyzing and perfecting the technology that has revolutionized gaming graphics. "We have a big supercomputer at NVIDIA that is running 24/7, 365 days a year improving DLSS," Catanzaro explained during his presentation on DLSS 4. The supercomputer's primary task involves analyzing failures in DLSS performance, such as ghosting, flickering, or blurriness across hundreds of games. When issues are identified, the system augments its training data sets with new examples of optimal graphics and challenging scenarios that DLSS needs to address.
DLSS 4 is the first move from convolutional neural networks to a transformer model that runs locally on client PCs. The continuous learning process has been crucial in refining the technology, with the dedicated supercomputer serving as the backbone of this evolution. The scale of resources allocated to DLSS development is massive, as the entire pipeline for a self-improving DLSS model must consist of not only thousands but tens of thousands of GPUs. Of course, a company making 100,000 GPU data centers (xAI's Colossus) must save some for itself and is proactively using it to improve its software stack. NVIDIA's CEO Jensen Huang famously said that DLSS can predict the future. Of course, these statements are to be tested when the Blackwell series launches. However, the approach of using massive data centers to improve DLSS is quite interesting, and with each new GPU generation NVIDIA release, the process is getting significantly sped up.
Source:
via PC Gamer
DLSS 4 is the first move from convolutional neural networks to a transformer model that runs locally on client PCs. The continuous learning process has been crucial in refining the technology, with the dedicated supercomputer serving as the backbone of this evolution. The scale of resources allocated to DLSS development is massive, as the entire pipeline for a self-improving DLSS model must consist of not only thousands but tens of thousands of GPUs. Of course, a company making 100,000 GPU data centers (xAI's Colossus) must save some for itself and is proactively using it to improve its software stack. NVIDIA's CEO Jensen Huang famously said that DLSS can predict the future. Of course, these statements are to be tested when the Blackwell series launches. However, the approach of using massive data centers to improve DLSS is quite interesting, and with each new GPU generation NVIDIA release, the process is getting significantly sped up.
62 Comments on NVIDIA Reveals Secret Weapon Behind DLSS Evolution: Dedicated Supercomputer Running for Six Years
especially if it’s about a topic I’m at home at.
i think it’s going to become very bland very quickly playing ai generated stories.
FSR 4.0 is going to be very interesting.
>Proceeds to praise an attempt at doing the same thing from the competitor because you like them
The hallmark of the average AMD fan
The "real-time" part that runs on the gpu is what's called inference, where you run a model that was trained to do something.
The training part often takes way longer, and you need to keep iterating on it as time goes in order to improve its performance over time.
It just so far the capabilities of AI was limited by hardware running the models.
Now we have come to the point that AI can use the “new” current hardware to improve everything. Models, algorithms, data acquisition and even it self. Large supercomputers are needed still just like 40-50 years ago in order to have the compute power that now we all have in our pockets.
The real breakthrough will come once compute power grows exponentially. Something else than today’s silicon based chips. The wall of improvement is coming fast and for next years AI/ML alone is the way around it with these “new” hardware that will keep growing in quantities.
Quantum computers is one strong candidate for replacing current tech. Just one of them will be able to replace entire rooms of servers. But it’s not a tech for personal/individual usage as it requires isolated near absolute zero conditions to work properly without any “outside” particle interference.
This type of compute power will improve AI vastly.
At some point, after them, almost everything will be cloud serviced. In the mean time IoT is going to continue to evolve as it is required as the base infrastructure for all this to happen.
One example is that cars will be all connected to each other and cross talk.
Tesla’s self driving software up to v11 was code written by men. Hundreds of of thousands of code lines. From v12+ AI took over and now there is no need for men to write any code. It acquires all data from existing human driven vehicles on the road, asses them into safe and unsafe based on the after come results, and use the “best” to improve the model, in a nutshell.
If you see how the older (pre v12) versions behave in comparison with the latest, the difference is night and day. And this is improved fast by every single step.
I’m not trying to paint an all pink happy cloud picture. Just stating the obvious. Like anything else humans ever created it has the goods and the bads.
Every aspect (good/bad) though will grow exponentially just like AI itself.
There are people and teams that are working daily to predict the (positive/negative) implications upon the society. It’s not a simple matter at all.
Unless someone explain it to us we can’t even begin to imagine the potential (positive/negative) impact.
And be assured that on the opposite side there are teams that researching how to exploit the negatives just like anything else.
I do try to keep up with subject.
Reel your neck in and mute me so I don't have to deal with your crazy unhinged religious attacks like this.
You come across as some kind of amateur bully boy, attacking anyone who doesn't agree with your religious beliefs, you should see what my comment was saying is that how come nv needs allegedly 6 years of supercomputing time, when FSR and Sony's PSSR is supposed to use on-chip A.I. to render its frames? I offer the possibility that it's only to lock devs into nv's propriety algorithms, which they have to pay for.
Take a quick look at how FSR works as an example. FSR 1 started as a simple CAS shader, you could load it through ReShade on any GPU from any vendor before AMD even added it tô games.
It eventually grew into a more complex upscaling solution but it never leveraged AI or matrix multiplication, not because they are nice or zeal for openness but because AMD's hardware is the only one in the industry which is not capable of it. And FSR 4, which allegedly does leverage machine learning algorithms, will be gated to the RX 9070 series, so much for that defense of open compatibility.
PSSR, as everything Sony, is fully proprietary, poorly documented to the public and apparently has been relatively poorly received so far. I don't believe it has any particular need for ML hardware since the PS5 Pro's graphics are still based on RDNA 2, which does not have this capability. Unless there is a semicustom solution, but I don't believe this to be the case.
Meanwhile, DLSS has been an ML trained model designed to reconstruct the image from less pixels from the very start, when it was introduced 7 years ago alongside the RTX 20 series.
The same applies to XeSS 1, but Intel went a step beyond and allowed it to run (albeit much slower) on any hardware they supports DP4A instructions. Which includes Nvidia Pascal and newer, but excludes RX Vega (exception of Radeon VII) and the original RDNA architecture (5700 XT).
I might have come off as harsh (yes I'll take the blame for it), and apologize if there was genuinely no malice in your initial remarks.
What this supercomputer does is separate of what an individual GPU is doing on the end user PC. This "server" simulates gaming on a wide variety of games and searching to find image errors after the upscaling and DLSS application. Then it tries to improve the model of the DLSS reconstruction. Every new version of DLSS reconstruction model with new enhancements is distributed through drivers to all end users.
So the reconstruction model is indeed running locally on every GPU but on the background the server is keep improving it.
How is that?
Essentially correct. Each local users GPU uses a model that was created on that supercomputer. I assume the improvements are delivered via updates to DLSS profiles which the NV driver searches for and pulls when launching a DLSS enabled title (it does that). Works both ways, from how they word it - PCs with telemetry enabled send back the information on what I assume are considered errors and weak points of the model usage in each supported title to facilitate improvements. They ARE being somewhat vague on what EXACTLY is done behind the scenes.
During the inference stage, the model will then be run, producing predictions and conclusions based on the data from the training stage, thus maximizing the performance of the AI model as all available computing power will be used to apply the model instead of thinking about how it should work.
It's generative AI 101
"The supercomputer's primary task involves analyzing failures in DLSS performance, such as ghosting, flickering, or blurriness across hundreds of games. When issues are identified, the system augments its training data sets with new examples of optimal graphics and challenging scenarios that DLSS needs to address."
Sounds like it runs and resets simulation gaming to continuously improve the model of prediction.
The stuff JHH showed at CES is what allowed full scene pathtracing to be even feasible.
I can see all the various problems playing Cyberpunk for instance, and the problems do not seem to get any better with newer DLSS DLLs. So why is it not better now than it was 2 years ago?
I'm not bashing nv personally, I do not use AMD graphics, and likely never will. But all this A.I. supercomputer nonsense makes me annoyed because it's a term that's just plastered all over everything and is 99.9% of the time, a complete lie. I think if A.I. was as advanced as nv claims, then why are they still using a remote supercomputer to render DLSS and not locally on the card itself?
I have heard that AMD are using pure A.I. in its upcoming FSR 4, and Sony is already using it on the PS5 Pro. And for the record, I'm not bashing DLSS, I enjoy it and find it a plus to my old RTX2070!
The individual end user GPU is running the model of reconstruction and prediction on the game that the user is running. It doesn't improve the model of reconstruction/prediction, only runs it.
The model improvement is done be the server. They are not necessarily communicate with each other on the fly.
What you're implying is akin to considering the DirectX DLLs to basically contain all the code necessary to display any game on their own, for example.
Without the software providing such updated training data for inference, updating the runtime is of questionable benefit at best. That's why updating the DLSS DLL won't increase image quality, it might improve performance ever so slightly - and even then, that is also anecdotal.
You might get a small improvement, you might not. Anything of quantifiable substance will require updated data which can only come with a software update.
You want a good AA technique? Just have a look on how older games were looking with 4xSSAA or even 8xSSAA.
Those were the best times.