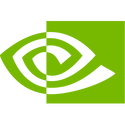
US Bans Export of NVIDIA H20 Accelerators to China, a Potential $5.5 Billion Loss for NVIDIA
President Trump's administration has announced that NVIDIA's H20 AI chip will require a special export license for any shipment to China, Hong Kong, or Macau for the indefinite future. The Commerce Department delivered the news to NVIDIA on April 14, 2025, citing worries that the H20 could be redirected into Chinese supercomputers with potential military applications. NVIDIA designed the H20 specifically to comply with earlier US curbs by scaling back performance from its flagship H100 model. The H20 features 96 GB of HBM3 memory running at up to 4.0 TB/s, delivers roughly 296 TeraFLOPS of mixed‑precision compute power, and offers a performance density of about 2.9 TeraFLOPS per die. Its single‑precision (FP32) throughput is around 74 TeraFLOPS, with FP16 performance reaching approximately 148 TeraFLOPS. In a regulatory filing on April 15, NVIDIA warned that it will record about $5.5 billion in writedowns this quarter related to H20 inventory and purchase commitments now blocked by the license requirement.
Shares of NVIDIA fell roughly 6 percent in after‑hours trading on April 15, triggering a wider sell‑off in semiconductor stocks from the US to Japan. South Korea's Samsung and SK Hynix each slid about 3 percent, while AMD also dropped on concerns about broader chip‑export curbs. Analysts at Bloomberg Intelligence project that, if the restrictions persist, NVIDIA's China‑related data center revenue could shrink to low‑ or mid‑single digits as a percentage of total sales, down from roughly 13 percent in fiscal 2024. Chinese AI players such as Huawei stand to gain as customers seek alternative inference accelerators. Commerce Secretary Howard Lutnick has pledged to maintain a tough stance on chip exports to China even as NVIDIA commits up to $500 billion in US AI infrastructure investments over the next four years. Everyone is now watching closely to see whether any H20 export licenses are approved and how long the ban might remain in place.
Shares of NVIDIA fell roughly 6 percent in after‑hours trading on April 15, triggering a wider sell‑off in semiconductor stocks from the US to Japan. South Korea's Samsung and SK Hynix each slid about 3 percent, while AMD also dropped on concerns about broader chip‑export curbs. Analysts at Bloomberg Intelligence project that, if the restrictions persist, NVIDIA's China‑related data center revenue could shrink to low‑ or mid‑single digits as a percentage of total sales, down from roughly 13 percent in fiscal 2024. Chinese AI players such as Huawei stand to gain as customers seek alternative inference accelerators. Commerce Secretary Howard Lutnick has pledged to maintain a tough stance on chip exports to China even as NVIDIA commits up to $500 billion in US AI infrastructure investments over the next four years. Everyone is now watching closely to see whether any H20 export licenses are approved and how long the ban might remain in place.