Monday, December 23rd 2024
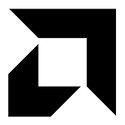
AMD's Pain Point is ROCm Software, NVIDIA's CUDA Software is Still Superior for AI Development: Report
The battle of AI acceleration in the data center is, as most readers are aware, insanely competitive, with NVIDIA offering a top-tier software stack. However, AMD has tried in recent years to capture a part of the revenue that hyperscalers and OEMs are willing to spend with its Instinct MI300X accelerator lineup for AI and HPC. Despite having decent hardware, the company is not close to bridging the gap software-wise with its competitor, NVIDIA. According to the latest report from SemiAnalysis, a research and consultancy firm, they have run a five-month experiment using Instinct MI300X for training and benchmark runs. And the findings were surprising: even with better hardware, AMD's software stack, including ROCm, has massively degraded AMD's performance.
"When comparing NVIDIA's GPUs to AMD's MI300X, we found that the potential on paper advantage of the MI300X was not realized due to a lack within AMD public release software stack and the lack of testing from AMD," noted SemiAnalysis, breaking down arguments in the report further, adding that "AMD's software experience is riddled with bugs rendering out of the box training with AMD is impossible. We were hopeful that AMD could emerge as a strong competitor to NVIDIA in training workloads, but, as of today, this is unfortunately not the case. The CUDA moat has yet to be crossed by AMD due to AMD's weaker-than-expected software Quality Assurance (QA) culture and its challenging out-of-the-box experience."NVIDIA has a massive advantage in that the software is fully functional. "As fast as AMD tries to fill in the CUDA moat, NVIDIA engineers are working overtime to deepen said moat with new features, libraries, and performance updates," noted the SemiAnalysis report. Tinybox and Tinybox Pro developer Tinygrad also confirmed this multiple times on their X profile, which also had a massive issue with AMD software in the past.
When taking a look at AMD Instinct MI300X and NVIDIA H100/H200 chips from 2023, the MI300X emerges as a clear winner performance-wise. It reaches 1,307 TFLOP/s for FP16 calculations, surpassing NVIDIA's H100, which delivers 989 TFLOP/s. The MI300X has 192 GB of HBM3 memory and a memory bandwidth of 5.3 TB/s. These specifications are even favourable to NVIDIA's H200, which offers 141 GB of HBM3e memory and 4.8 TB/s of memory bandwidth. The AMD chip also even has a lower total cost of ownership model, which has a 40% cheaper networking alone. On paper, the AMD chip looks superior to NVIDIA's Hopper offerings, but in reality, not so much.
AMD's internal teams have little access to GPU boxes to develop and refine the ROCm software stack. Tensorwave, which is among the largest providers of AMD GPUs in the cloud, took their own GPU boxes and gave AMD engineers the hardware on demand, free of charge, just so the software could be fixed. This is all while Tensorwave paid for AMD GPUs, renting their own GPUs back to AMD free of charge. Finally, SemiAnalysis has noted that the AMD software stack has been improved based on their suggestions. Still, there is a long way to go before the company reaches NVIDIA's CUDA level of stability and performance. For detailed analysis, visit SemiAnalysis report here.
Source:
SemiAnalysis
"When comparing NVIDIA's GPUs to AMD's MI300X, we found that the potential on paper advantage of the MI300X was not realized due to a lack within AMD public release software stack and the lack of testing from AMD," noted SemiAnalysis, breaking down arguments in the report further, adding that "AMD's software experience is riddled with bugs rendering out of the box training with AMD is impossible. We were hopeful that AMD could emerge as a strong competitor to NVIDIA in training workloads, but, as of today, this is unfortunately not the case. The CUDA moat has yet to be crossed by AMD due to AMD's weaker-than-expected software Quality Assurance (QA) culture and its challenging out-of-the-box experience."NVIDIA has a massive advantage in that the software is fully functional. "As fast as AMD tries to fill in the CUDA moat, NVIDIA engineers are working overtime to deepen said moat with new features, libraries, and performance updates," noted the SemiAnalysis report. Tinybox and Tinybox Pro developer Tinygrad also confirmed this multiple times on their X profile, which also had a massive issue with AMD software in the past.
When taking a look at AMD Instinct MI300X and NVIDIA H100/H200 chips from 2023, the MI300X emerges as a clear winner performance-wise. It reaches 1,307 TFLOP/s for FP16 calculations, surpassing NVIDIA's H100, which delivers 989 TFLOP/s. The MI300X has 192 GB of HBM3 memory and a memory bandwidth of 5.3 TB/s. These specifications are even favourable to NVIDIA's H200, which offers 141 GB of HBM3e memory and 4.8 TB/s of memory bandwidth. The AMD chip also even has a lower total cost of ownership model, which has a 40% cheaper networking alone. On paper, the AMD chip looks superior to NVIDIA's Hopper offerings, but in reality, not so much.
AMD's internal teams have little access to GPU boxes to develop and refine the ROCm software stack. Tensorwave, which is among the largest providers of AMD GPUs in the cloud, took their own GPU boxes and gave AMD engineers the hardware on demand, free of charge, just so the software could be fixed. This is all while Tensorwave paid for AMD GPUs, renting their own GPUs back to AMD free of charge. Finally, SemiAnalysis has noted that the AMD software stack has been improved based on their suggestions. Still, there is a long way to go before the company reaches NVIDIA's CUDA level of stability and performance. For detailed analysis, visit SemiAnalysis report here.
33 Comments on AMD's Pain Point is ROCm Software, NVIDIA's CUDA Software is Still Superior for AI Development: Report
Finally, all hardware companies need to become software companies. Engineers and black box management are stuck in the past.
Edit: oh and the article didn’t say moat enough…moat.
Maybe if their share price drops down to $50?
But I will admit, it sounds way to crazy to be real.
Funny enough, I bumped into this:
blog.runpod.io/amd-mi300x-vs-nvidia-h100-sxm-performance-comparison-on-mixtral-8x7b-inference/
And someone that do work with MI300 hardware and ROCm, posted this:
Mere coincidence that both use the appropriate name Ngreedia. :D
Funny enough, at some point I believe AMD hardware was actually superior when it came to compute. However, what matters is the complete stack.
This both backs up the analysis as well as shows distortion by not giving the full picture.
AMD needs to work on software to gain competitiveness on training, and there may be architectural limitations that cap its overall training performance (xGMI interconnect arch)
They definitely need better regression testing and testing in general. They have acquired several Ai software companies this year that may help with this.
So the current reality is...
If you are using off the shelf models mi300x excels, if you finetune those models, AMD excels, If you train from scratch... AMD kinda sucks.
The analysis also fails to grasp the reality of availability... sometimes its better to have not as good than nothing.
We all agree Lisa Su is competent, correct? Do any of us actually believe that people are telling her: "We need to do better with our software" and she's like "Ahhh, screw it"?
So what is it then? I imagine it's difficult for them to get ahold and maintain talent, Nvidia and Intel can afford to pay them more, and both competitors have far larger R&D budgets, is that the problem? Is it a workplace "culture" problem? It'd be amazing to hear from someone who has worked there to see if that's the case... If anyone has some educated and informed guesses, I'd love to hear them, because it surely cannot be that AMD is just being "stupid" or something.....but there definitely is a problem or problems
>>...Is it a workplace "culture" problem? It'd be amazing to hear from someone who has worked there to see if that's the case...
I worked for AMD as a contractor. I have very-very good memories for just a couple of fellow developers. No any good memories for the management of AMD. In overall: The Environment inside of AMD is Very Toxic.
>>...AMD's software stack, including ROCm, has massively degraded AMD's performance...
Worked with ROCm a lot and I would rate ROCm as A-Piece-of-Over-Complecated-Software-Crap.
>>...MI300X was not realized due to a lack within AMD public release software stack and the lack of testing from AMD...
Not true based on my experience however it is possible things have changed after my contract was over.
>>...AMD's software experience is riddled with bugs rendering out of the box training with AMD is impossible...
Partially true since I was able to see how a lot of bugs were Not fixed at all.
>>...We were hopeful that AMD could emerge as a strong competitor to NVIDIA
Not possible due to internal problems with retaining very experienced C/C++ software engineers.
>>...AMD's weaker-than-expected software Quality Assurance (QA) culture and its challenging out-of-the-box experience...
Very surprised to read about it since QA was Very Strong when I was working for AMD. It is possible things have changed after my contract was over.
>>...AMD's internal teams have little access to GPU boxes to develop and refine the ROCm software stack...
Absolutely surprised to read about it. Once again, it is possible things have changed...
No no, they also suck at the thing they didn't cover...
They used off the shelf containers, to train.... not pretrained models to infer.
There is a reason Meta uses H100/200 to train and uses mi300x to infer.
From the article (I'm still reading it):
This contrasts with Nvidia’s NCCL team, which has access to R&D resources on Nvidia’s 11,000 H100 internal EOS cluster. Furthermore, Nvidia has Sylvain Jeaugey, who is the subject matter expert on collective communication. There are a lot of other world class collective experts working at Nvidia as well, and, unfortunately, AMD has largely failed to attract collective library talent due to less attractive compensation and resources – as opposed to engineers at Nvidia, where it is not uncommon to see engineers make greater than a million dollars per year thanks to appreciation in the value of RSUs. This is true:
Another core reason for this problem is that the lead maintainer of PyTorch (Meta) does not currently use MI300X internally for production LLM training, leading to code paths not used internally at Meta being buggy and not dogfooded properly. We believe AMD should partner with Meta to get their internal LLM training working on MI300X.
x.com/x/status/1870498560820867482
www.techpowerup.com/vgabios/219429/219429
[ICODE]Vega10 A1 XT AIR D05011 8GB 852e/945m 1.0V SWQA[/ICODE]
This was intended for debugging the Vega FE without having access to the hardware itself by converting the regular gaming RX Vega 64 into one. It was leaked to TPU by a (likely disgruntled) AMD employee in back in March of 2020. To be fair, it becomes functionally identical, other than the halved VRAM capacity, but I still think it is absolutely mind blowing that even their driver developers do not have crates of cards at their disposal, so they can test every wild variant out there. Something no doubt both Intel and NVIDIA provided their software engineers with.
There's plenty decent people working hard, just not in the right places.
It is super super easy to run models on mi100/250x/300x 7900gre/xt/xtx
I read as far as the paywall goes.
I also... have used ROCm since vega64/mi25
I also... have used Cuda since K80/GTX690
I currently run a hive of mi100s, and a sxm v100 box.
When it comes to inference, MI300x gets day0 support. Training is very lacking and Nvidia's deep bench of software engineers shows.
I expect part 2 of the article to be a bit different.
I am fully aware of the lacking's of AMDs ecosystem, but I am also aware of its strengths.
And the ability to just grab containers and go exists... hugging face is full of native containers for ROCm, Hipify can convert most* things that are cuda native, abet at performance penalty.
But when it comes to inference AMD is not a 2nd class citizen. It has full support with triton, and flash attention...
And Llama 405b fp16 launched exclusively on mi300x, most likely due to the ram requirements.
As it was quantized down to fp8, then it could fit on 8x h100 80gb, but as it was announced, Meta and AMD announced together that all Meta 405b live instances were run on mi300x.
If that is still true or was just a limited exclusivity while it was quantized down... idk...
But claiming things like a mi300x cant run OOB models is just... ignorant af, and not even what the article claims.
It claims bad training performance and strange bugs and as a user of the ecosystem... yup. AMD has strange bugs.
They have known lockups for multi gpu instances... and the solution is to run additional grub parameters, perfectly stable with iommu=pt, randomly hangs without.
But all this information is in the tuning guides. The install process is easy, and hugging face is full of models to run.
Later.