Monday, November 17th 2014
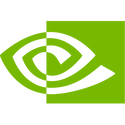
NVIDIA Unveils Tesla K80 Dual-Chip Compute Accelerator
NVIDIA today unveiled a new addition to the NVIDIA Tesla Accelerated Computing Platform: the Tesla K80 dual-GPU accelerator, the world's highest performance accelerator designed for a wide range of machine learning, data analytics, scientific, and high performance computing (HPC) applications.
The Tesla K80 dual-GPU is the new flagship offering of the Tesla Accelerated Computing Platform, the leading platform for accelerating data analytics and scientific computing. It combines the world's fastest GPU accelerators, the widely used CUDA parallel computing model, and a comprehensive ecosystem of software developers, software vendors, and datacenter system OEMs.The Tesla K80 dual-GPU accelerator delivers nearly two times higher performance and double the memory bandwidth of its predecessor, the Tesla K40 GPU accelerator. With ten times higher performance than today's fastest CPU, it outperforms CPUs and competing accelerators on hundreds of complex analytics and large, computationally intensive scientific computing applications.
Users can unlock the untapped performance of a broad range of applications with the accelerator's enhanced version of NVIDIA GPU Boost technology (PDF), which dynamically converts power headroom into the optimal performance boost for each individual application.
Industry-Leading Performance for Science, Data Analytics, Machine Learning
The Tesla K80 dual-GPU accelerator was designed with the most difficult computational challenges in mind, ranging from astrophysics, genomics and quantum chemistry to data analytics. It is also optimized for advanced deep learning tasks, one of the fastest growing segments of the machine learning field.
"NVIDIA GPUs have become the de facto computing platform for the deep learning community," said Yann LeCun, director of AI Research at Facebook, and Silver Professor of Computer Science & Neural Science at New York University. "Because the accuracy of deep learning systems improves as the models and datasets get larger, we always look for the fastest hardware we can find. The Tesla K80 accelerator, with its dual-GPU architecture and large memory, gives us more teraflops and more GB than ever before from a single server, allowing us to make faster progress in deep learning."
The Tesla K80 delivers up to 8.74 teraflops single-precision and up to 2.91 teraflops double-precision peak floating point performance, and10 times higher performance than today's fastest CPUs on leading science and engineering applications, such as AMBER, GROMACS, Quantum Espresso and LSMS.
"The Tesla K80 dual-GPU accelerators are up to 10 times faster than CPUs when enabling scientific breakthroughs in some of our key applications, and provide a low energy footprint," said Wolfgang Nagel, director of the Center for Information Services and HPC at Technische Universität Dresden in Germany. "Our researchers use the available GPU resources on the Taurus supercomputer extensively to enable a more refined cancer therapy, understand cells by watching them live, and study asteroids as part of ESA's Rosetta mission."
Key features of the Tesla K80 dual-GPU accelerator include:
More information about the Tesla K80 dual-GPU accelerator is available at NVIDIA booth 1727 at SC14, Nov. 17-20, and on the NVIDIA high performance computing website.
Users can also try the Tesla K80 dual-GPU accelerator for free on remotely hosted clusters. Visit the GPU Test Drive website for more information.
Availability
Shipping today, the NVIDIA Tesla K80 dual-GPU accelerator will be available from a variety of server manufacturers, including ASUS, Bull, Cirrascale, Cray, Dell, Gigabyte, HP, Inspur, Penguin, Quanta, Sugon, Supermicro and Tyan, as well as from NVIDIA reseller partners.
The Tesla K80 dual-GPU is the new flagship offering of the Tesla Accelerated Computing Platform, the leading platform for accelerating data analytics and scientific computing. It combines the world's fastest GPU accelerators, the widely used CUDA parallel computing model, and a comprehensive ecosystem of software developers, software vendors, and datacenter system OEMs.The Tesla K80 dual-GPU accelerator delivers nearly two times higher performance and double the memory bandwidth of its predecessor, the Tesla K40 GPU accelerator. With ten times higher performance than today's fastest CPU, it outperforms CPUs and competing accelerators on hundreds of complex analytics and large, computationally intensive scientific computing applications.
Users can unlock the untapped performance of a broad range of applications with the accelerator's enhanced version of NVIDIA GPU Boost technology (PDF), which dynamically converts power headroom into the optimal performance boost for each individual application.
Industry-Leading Performance for Science, Data Analytics, Machine Learning
The Tesla K80 dual-GPU accelerator was designed with the most difficult computational challenges in mind, ranging from astrophysics, genomics and quantum chemistry to data analytics. It is also optimized for advanced deep learning tasks, one of the fastest growing segments of the machine learning field.
"NVIDIA GPUs have become the de facto computing platform for the deep learning community," said Yann LeCun, director of AI Research at Facebook, and Silver Professor of Computer Science & Neural Science at New York University. "Because the accuracy of deep learning systems improves as the models and datasets get larger, we always look for the fastest hardware we can find. The Tesla K80 accelerator, with its dual-GPU architecture and large memory, gives us more teraflops and more GB than ever before from a single server, allowing us to make faster progress in deep learning."
The Tesla K80 delivers up to 8.74 teraflops single-precision and up to 2.91 teraflops double-precision peak floating point performance, and10 times higher performance than today's fastest CPUs on leading science and engineering applications, such as AMBER, GROMACS, Quantum Espresso and LSMS.
"The Tesla K80 dual-GPU accelerators are up to 10 times faster than CPUs when enabling scientific breakthroughs in some of our key applications, and provide a low energy footprint," said Wolfgang Nagel, director of the Center for Information Services and HPC at Technische Universität Dresden in Germany. "Our researchers use the available GPU resources on the Taurus supercomputer extensively to enable a more refined cancer therapy, understand cells by watching them live, and study asteroids as part of ESA's Rosetta mission."
Key features of the Tesla K80 dual-GPU accelerator include:
- Two GPUs per board - Doubles throughput of applications designed to take advantage of multiple GPUs.
- 24GB of ultra-fast GDDR5 memory - 12GB of memory per GPU, 2x more memory than Tesla K40 GPU, allows users to process 2x larger datasets.
- 480GB/s memory bandwidth - Increased data throughput allows data scientists to crunch though petabytes of information in half the time compared to the Tesla K10 accelerator. Optimized for energy exploration, video and image processing, and data analytics applications.
- 4,992 CUDA parallel processing cores - Accelerates applications by up to 10x compared to using a CPU alone.
- Dynamic NVIDIA GPU Boost Technology - Dynamically scales GPU clocks based on the characteristics of individual applications for maximum performance.
- Dynamic Parallelism - Enables GPU threads to dynamically spawn new threads, enabling users to quickly and easily crunch through adaptive and dynamic data structures.
More information about the Tesla K80 dual-GPU accelerator is available at NVIDIA booth 1727 at SC14, Nov. 17-20, and on the NVIDIA high performance computing website.
Users can also try the Tesla K80 dual-GPU accelerator for free on remotely hosted clusters. Visit the GPU Test Drive website for more information.
Availability
Shipping today, the NVIDIA Tesla K80 dual-GPU accelerator will be available from a variety of server manufacturers, including ASUS, Bull, Cirrascale, Cray, Dell, Gigabyte, HP, Inspur, Penguin, Quanta, Sugon, Supermicro and Tyan, as well as from NVIDIA reseller partners.
10 Comments on NVIDIA Unveils Tesla K80 Dual-Chip Compute Accelerator
I love the design of these Tesla cards, just fantastic looking crafted and refined work horses!
Source: videocardz.com
I thought that Nvidia designated that chips with defects get sold as GeForce products. Since GK210 doesn't seem like it will ever make it to a GeForce card and since yields will never be great on a ~600mm^2 chip then they would have to use harvested silicon or face very low yields/high costs.
This seems aimed squarely at Nvidia's contribution to OpenPOWER (NVLink included). Look at the launch partners (Cray, Dell, HP, Quanta), the timing (both at SC14) WRT to Knights Landing/Knights Hill and POWER9, and who owns the non-3D big data space (IBM). SC14 is basically an exercise in muscle flexing for three consortiums.