Wednesday, October 3rd 2018
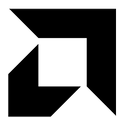
AMD and Xilinx Announce a New World Record for AI Inference
At today's Xilinx Developer Forum in San Jose, Calif., our CEO, Victor Peng was joined by the AMD CTO Mark Papermaster for a Guinness. But not the kind that comes in a pint - the kind that comes in a record book. The companies revealed the AMD and Xilinx have been jointly working to connect AMD EPYC CPUs and the new Xilinx Alveo line of acceleration cards for high-performance, real-time AI inference processing. To back it up, they revealed a world-record 30,000 images per-second inference throughput!
The impressive system, which will be featured in the Alveo ecosystem zone at XDF today, leverages two AMD EPYC 7551 server CPUs with its industry-leading PCIe connectivity, along with eight of the freshly-announced Xilinx Alveo U250 acceleration cards. The inference performance is powered by Xilinx ML Suite, which allows developers to optimize and deploy accelerated inference and supports numerous machine learning frameworks such as TensorFlow. The benchmark was performed on GoogLeNet, a widely used convolutional neural network.AMD and Xilinx have shared a common vision around the evolution of computing to heterogeneous system architecture and have a long history of technical collaboration. Both companies have optimized drivers and tuned the performance for interoperability between AMD EPYC CPUs with Xilinx FPGAs. We are also collaborating with others in the industry on cache coherent interconnect for accelerators (the CCIX Consortium - pronounced "see-six"), focused on enabling cache coherency and shared memory across multiple processors.
AMD EPYC is the perfect CPU platform for accelerating artificial intelligence and high- performance computing workloads. With 32 cores, 64 threads, 8 memory channels with up to 2 TB of memory per socket, and 128 PCIe lanes coupled with the industry's first hardware-embedded x86 server security solution, EPYC is designed to deliver the memory capacity, bandwidth, and processor cores to efficiently run memory-intensive workloads commonly seen with AI and HPC. With EPYC, customers can collect and analyze larger data sets much faster, helping them significantly accelerate complex problems.
Xilinx and AMD see a bright future in their technology collaboration. There is strong alignment in our roadmaps that align the high-performance AMD EPYC server and graphics processors with Xilinx acceleration platforms across its Alveo accelerator cards, as well as its forthcoming Versal portfolio.
So, raise a pint to the future of AI inference and innovation for heterogeneous computing platforms. And don't forget to stop by and see the system in action in the Alveo ecosystem zone at the Fairmont hotel.
The impressive system, which will be featured in the Alveo ecosystem zone at XDF today, leverages two AMD EPYC 7551 server CPUs with its industry-leading PCIe connectivity, along with eight of the freshly-announced Xilinx Alveo U250 acceleration cards. The inference performance is powered by Xilinx ML Suite, which allows developers to optimize and deploy accelerated inference and supports numerous machine learning frameworks such as TensorFlow. The benchmark was performed on GoogLeNet, a widely used convolutional neural network.AMD and Xilinx have shared a common vision around the evolution of computing to heterogeneous system architecture and have a long history of technical collaboration. Both companies have optimized drivers and tuned the performance for interoperability between AMD EPYC CPUs with Xilinx FPGAs. We are also collaborating with others in the industry on cache coherent interconnect for accelerators (the CCIX Consortium - pronounced "see-six"), focused on enabling cache coherency and shared memory across multiple processors.
AMD EPYC is the perfect CPU platform for accelerating artificial intelligence and high- performance computing workloads. With 32 cores, 64 threads, 8 memory channels with up to 2 TB of memory per socket, and 128 PCIe lanes coupled with the industry's first hardware-embedded x86 server security solution, EPYC is designed to deliver the memory capacity, bandwidth, and processor cores to efficiently run memory-intensive workloads commonly seen with AI and HPC. With EPYC, customers can collect and analyze larger data sets much faster, helping them significantly accelerate complex problems.
Xilinx and AMD see a bright future in their technology collaboration. There is strong alignment in our roadmaps that align the high-performance AMD EPYC server and graphics processors with Xilinx acceleration platforms across its Alveo accelerator cards, as well as its forthcoming Versal portfolio.
So, raise a pint to the future of AI inference and innovation for heterogeneous computing platforms. And don't forget to stop by and see the system in action in the Alveo ecosystem zone at the Fairmont hotel.
24 Comments on AMD and Xilinx Announce a New World Record for AI Inference
Each card has two Quad Small Form-factor Pluggable fiber links at 100 Gb each. Why?
Alveo U250 Data Center Accelerator Card
At the heart of the Xilinx Alveo U200 and U250 accelerator cards are custom-built UltraScale+ FPGAs that run or optimally (and exclusively) on Alveo .
It's FPGA-based, so the chip is designed precisely for inference. It's a few times faster than a GPU would be (4x faster than V100, graph below).
It's important to state that the actual Xilinx product is the Alveo accelerator and it's performing the inference tasks. CPUs are here just to run the platform and push data around. It might as well be using Xeons.
It was most likely AMD's initiative to be mentioned here.
It may have been an easy decision for Xilinx, since their main competitor is Intel as well (Stratix-based accelerator was launched just a few days ago).
As for performance, here's a comparison to some alternatives from a Xilinx whitepaper.
www.xilinx.com/support/documentation/white_papers/wp504-accel-dnns.pdf
And now the fun part
Arria-10 is a competing FPGA product... but not the latest one from Altera/Intel. Xilinx says:
"3. Arria-10 numbers taken Intel White Paper, "Accelerating Deep Learning with the OpenCL™ Platform and Intel Stratix 10 FPGAs."
builders.intel.com/docs/aibuilders/accelerating-deep-learning-with-the-opencl-platform-and-intel-stratix-10-fpgas.pdf."
But you know what's also in this white paper? Surprise... it's Stratix 10 performance! :-D
Also, inference is not exactly a material for parallelism.
Multi-thread gain here is mostly done by batching, i.e. you're performing calculations on many samples at the same time.
Maybe these ports can be used to access the cards in a "cluster" if there aren't enough PCIe slots? Even this is a stretch, though.
Ordering Information
Ordering Contact Engineering Sample Contact an Intel® sales representative
OEM Partner Server Model Hewlett Packard Enterprise (HPE) Available 1H 2019
www.tomshardware.co.uk/intel-stratix-10-tx-fpga,news-57969.html
What you're thinking about is the latest Intel-built accelerator.
Also, have to wonder about the FP16/FP32 note on V100. Wasn't V100 capable of INT8 inferencing? :)
With that disclaimer out of the way, perhaps the application these cards run manages to take full advantage of the card's capabilities while that doesn't happen with nVidia cards?
These cards are out wrecking shop in the mining world as well posting huge numbers. I don't know who said the stratix 10 isn't out I have actually like held one in my hands and stuff a couple months back almost purchased a set, but it requires a much higher level of programming to set up than I was willing to put in.
Still, since on paper the difference is about 50% VS 500% actual difference ... that's a whole order of magnitude there ... something's not right, right?
BTW, not to knock the white paper, but if it actually is night and day faster, how could the record have been broken? From the whitepaper, Stratix should have it with half the cards in anybody's system.
130ns between dies on the same socket, and 250ns between dies on opposing sockets... so card 1 to card 2 is 130ns... card 1 to card 4 is 250ns, and card 2 to card 4 is 380ns... So long as things don't have to talk to each other, and you aren't hanging nvme as well... you can survive without a switch, otherwise you will quickly saturate the internal gmi and xgmi interconnects. Those are idle latencies btw...
Rome should solve most of this by straight up doubling the pcie lanes, bumping the ram frequency and making a seperate interconnect for accelerators.
Epyc is very competitive, but not without weakness.