Thursday, May 14th 2020
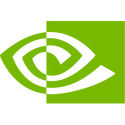
NVIDIA Ampere A100 Has 54 Billion Transistors, World's Largest 7nm Chip
Not long ago, Intel's Raja Koduri claimed that the Xe HP "Ponte Vecchio" silicon was the "big daddy" of Xe GPUs, and the "largest chip co-developed in India," larger than the 35 billion-transistor Xilinix VU19P FPGA co-developed in the country. It turns out that NVIDIA is in the mood for setting records. The "Ampere" A100 silicon has 54 billion transistors crammed into a single 7 nm die (not counting transistor counts of the HBM2E memory stacks).
NVIDIA claims a 20 Times boost in both AI inference and single-precision (FP32) performance over its "Volta" based predecessor, the Tesla V100. The chip also offers a 2.5X gain in FP64 performance over "Volta." NVIDIA has also invented a new number format for AI compute, called TF32 (tensor float 32). TF32 uses 10-bit mantissa of FP16, and the 8-bit exponent of FP32, resulting in a new, efficient format. NVIDIA attributes its 20x performance gains over "Volta" to this. The 3rd generation tensor core introduced with Ampere supports FP64 natively. Another key design focus for NVIDIA is to leverage the "sparsity" phenomenon in neural nets, to reduce their size, and improve performance.A new HPC-relevant feature being introduced with A100 is multi-instance GPU, which allows multiple complex applications to run on the same GPU without sharing resources such as memory bandwidth. The user can now partition a physical A100 into up to 7 virtual GPUs of varying specs, and ensure that an application running on one of the vGPUs doesn't eat into the resources of the other. As for real-world performance, NVIDIA claims that the A100 beat the V100 by a factor of 7 at BERT.
The DGX-A100 system crams 5 petaflops of compute peformance onto a single "graphics card" (a single node), and starts at $199,000 a piece.
Sources:
VideoCardz, EETimes
NVIDIA claims a 20 Times boost in both AI inference and single-precision (FP32) performance over its "Volta" based predecessor, the Tesla V100. The chip also offers a 2.5X gain in FP64 performance over "Volta." NVIDIA has also invented a new number format for AI compute, called TF32 (tensor float 32). TF32 uses 10-bit mantissa of FP16, and the 8-bit exponent of FP32, resulting in a new, efficient format. NVIDIA attributes its 20x performance gains over "Volta" to this. The 3rd generation tensor core introduced with Ampere supports FP64 natively. Another key design focus for NVIDIA is to leverage the "sparsity" phenomenon in neural nets, to reduce their size, and improve performance.A new HPC-relevant feature being introduced with A100 is multi-instance GPU, which allows multiple complex applications to run on the same GPU without sharing resources such as memory bandwidth. The user can now partition a physical A100 into up to 7 virtual GPUs of varying specs, and ensure that an application running on one of the vGPUs doesn't eat into the resources of the other. As for real-world performance, NVIDIA claims that the A100 beat the V100 by a factor of 7 at BERT.
The DGX-A100 system crams 5 petaflops of compute peformance onto a single "graphics card" (a single node), and starts at $199,000 a piece.
20 Comments on NVIDIA Ampere A100 Has 54 Billion Transistors, World's Largest 7nm Chip
Good catch! I noticed it too.
However, what's really going on is that they are creating their own alternative to BF16 (brainfloat16) and calling it TF32: keeping the 8 bits for exponent from fp32 but using only 10 bits for the fraction (precision) from fp16. This keeps the approximate range of FP32 while keeping the precision of FP16 (half precision). This is different than BF16 since BF16 only keeps 7 bits for precision. So, you can get better (or what some of my students say..."gooder") approximations with TF32 when converting back to FP32 (by padding the last 13 bits of precision on FP32 with zeroes) instead of with BF16 (where you would pad the last 16 bits with zeroes).
Does it really matter that much in the end? I'm not a professional with experience in AIs or DNNs but I suppose that FP32 approximations from TF32 is better and only ever so slightly slower than FP32 approximations from BF16. It is somewhat clever with what they did.
But, back to the whole TF19 bit (no pun intended!): I think it's a marketing move as TF32 "sounds" better. It's really TF19 with 1 bit sign, 8 bit exponent, and 10 bit fraction but, hey, "TF32" FTW. 7FF+ is supposedly on par with clock speeds or slightly better than 12FF. If it is indeed 8192 cores, then we'd have around 29.5 TFLOPS to 32.8 TFLOPS (1.8 GHz to 2 GHz, respectively). "TF32" could be as high as 655.4 TFLOPS, or, if one has a cool $200K lying around, you can get that monstrosity that JSH has been baking and get 8x 655.4 TFLOPS = 5.243 PFLOPS of "TF32" performance. I mean...saying PFLOPS like "Pee-Flops" is just ridiculous...
Aaaaaaand...I'm getting off topic.
tl;dr: Big dies are not the answer.
AMD have been scooping most of the biggest contracts lately, and most of the really big contracts in the last 18 months have been aiming at CDNA2 / Hopper, not Ampere or CDNA1.
That 20x figure is pure fantasy land.
Also i find it really funny that so many "home grown HPC experts" suddenly show up here claiming CDNA2 or whatever made-up crap.
HPC is all about ecosystem where both software and hardware are needed in excellent shape. Pay attention to how much Nvidia CEO acknowledged the software developers. Without a thriving software ecosystem, the hardware by themselves are nothing. In the field of AI, nobody is currently able to compete with Nvidia's software and hardware integration.
Computing hardware is only half (Hell, actually 1/3) of the deal. There is software which is a HUGE part, as well as inter-connecting hardware.
Get a clue.