Thursday, September 26th 2024
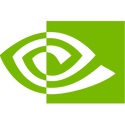
NVIDIA GeForce RTX 5090 and RTX 5080 Specifications Surface, Showing Larger SKU Segmentation
Thanks to the renowned NVIDIA hardware leaker kopite7Kimi on X, we are getting information about the final versions of NVIDIA's first upcoming wave of GeForce RTX 50 series "Blackwell" graphics cards. The two leaked GPUs are the GeForce RTX 5090 and RTX 5080, which now feature a more significant gap between xx80 and xx90 SKUs. For starters, we have the highest-end GeForce RTX 5090. NVIDIA has decided to use the GB202-300-A1 die and enabled 21,760 FP32 CUDA cores on this top-end model. Accompanying the massive 170 SM GPU configuration, the RTX 5090 has 32 GB of GDDR7 memory on a 512-bit bus, with each GDDR7 die running at 28 Gbps. This translates to 1,568 GB/s memory bandwidth. All of this is confined to a 600 W TGP.
When it comes to the GeForce RTX 5080, NVIDIA has decided to further separate its xx80 and xx90 SKUs. The RTX 5080 has 10,752 FP32 CUDA cores paired with 16 GB of GDDR7 memory on a 256-bit bus. With GDDR7 running at 28 Gbps, the memory bandwidth is also halved at 784 GB/s. This SKU uses a GB203-400-A1 die, which is designed to run within a 400 W TGP power envelope. For reference, the RTX 4090 has 68% more CUDA cores than the RTX 4080. The rumored RTX 5090 has around 102% more CUDA cores than the rumored RTX 5080, which means that NVIDIA is separating its top SKUs even more. We are curious to see at what price point NVIDIA places its upcoming GPUs so that we can compare generational updates and the difference between xx80 and xx90 models and their widened gaps.
Sources:
kopite7kimi (RTX 5090), kopite7kimi (RTX 5080)
When it comes to the GeForce RTX 5080, NVIDIA has decided to further separate its xx80 and xx90 SKUs. The RTX 5080 has 10,752 FP32 CUDA cores paired with 16 GB of GDDR7 memory on a 256-bit bus. With GDDR7 running at 28 Gbps, the memory bandwidth is also halved at 784 GB/s. This SKU uses a GB203-400-A1 die, which is designed to run within a 400 W TGP power envelope. For reference, the RTX 4090 has 68% more CUDA cores than the RTX 4080. The rumored RTX 5090 has around 102% more CUDA cores than the rumored RTX 5080, which means that NVIDIA is separating its top SKUs even more. We are curious to see at what price point NVIDIA places its upcoming GPUs so that we can compare generational updates and the difference between xx80 and xx90 models and their widened gaps.
185 Comments on NVIDIA GeForce RTX 5090 and RTX 5080 Specifications Surface, Showing Larger SKU Segmentation
The Titans had some appeal to prosumers because of the amount of VRAM. As you said the 3090 and 4090 just filled the slot that the Titan once held. You can think of the 3090 and 3090 Ti as something like the Kepler Titan and the Titan Black.
They are just making products with the above consumers in mind first, and then giving something "good enough" for gamers with a high markup, that most folks will pay anyway.
Most people here can complain and say they won't buy it, but Nvidia's revenue increase for their gaming sector tells that the actual market acts the other way around.
There will always be rich and poor people but people have to choice to vote with their wallets. And to be fair if people never bought GPUs at crazy high prices during the pandemic we would not be in this situation...
Also chip manufacturing and price of components keeps rising all the time so those companies won't stop charging more anytime soon.
But i'm sure not
Although the 5080 might cost the same and have all the new DLSS/FG and RT goodies that would work better and a revised more efficient thread engine.
There is also the possibility that the Blackwells will not be in supply enough to meet demand which leads to retailer price gouging as always. My plan is to wait about a year from now to pick up a 5090 after the dust settles so it was worth it to me to pick up a 4070 Super to hold me over until then. My 2070 Super, like yours, wasn't really cutting it at 1440p anymore and I made matters worse by deciding to give 4K a try which is great btw. All I have to do is put off playing any games that are GPU intensive for about a year.
If you don't want to wait on the 5070 you don't have to. You can pick up a 4070 Super and sell it later but as you know that decision will cost you a few hundred dollars.
Just power limit it to a reasonable value (~300W or so) and you're golden. Rumors still point out to it being a dual-slot GPU, idk how, but it'd be nice nonetheless.
Reminder that the 4090 also supports 600W configs, but most people don't use it like so. Saying that the 5090 will be a 600W GPU doesn't mean that it'll draw 600W all time.
But for a year or two it would be best available solution from the shelf - depend on scalpers demand.
edit
@igormp
edit2
In 5-6 years timeframe all soldered memory GPUs will be entry level class (focused on games) or obsolete imho.
Fiber connected memory large and modular banks will come to the mainstream at edge for machine learning tasks - its my prediction.
so 5090 with 32 or 48 GB dont exited me .
Even ROCm is still a pain to use on AMD GPUs.
Nvidia will likely still have the reign on that area for the next 5 years without issues. While the idea is nice, KANs are mathematically equivalent to your good old MLPs, and can also be run on GPUs.
I get your idea with "radically different alternatives", but your example is not one of those haha
I dont have on my mind of outer space solutions.:p
edit
semiengineering.com/hardware-acceleration-approach-for-kan-via-algorithm-hardware-co-design/
^ link to KAN implementation article ^ You are definitely overestimating AI bubble timeframe imho.
Yes they could reign but with quite new much energy efficient architectures = or they will die like dinos 65 mln years ago.
As I said before, KANs and MLP are mathematically equivalent, so you can implement KANs in ways that are more fitting to GPUs (as in matmuls) as well, while still maintaining the same capabilities of the original idea:
arxiv.org/abs/2406.02075v2
arxiv.org/abs/2408.11200 I could say the same for your ideas about decoupled memory, but I believe neither of us have a crystal ball, right?
Let me explain my point of view in details.
If you need let say 500 GB or 1 TB to run advanced LLM on your hardware so you dont want to get soldered them to a toy like 5090 which will have very limited lifespan for the sake of pace of changes in IC industry alone.
Decoupled memory is one time spending but its lifespan is twice or triple as long as lifespan of typical GPU .
If you dont belive me just look a check for how much gpu generations gddr5 or gddr6 were coupled.
So I assume the same will be valid for decoupled memories too - they will fit for many gpu generations 3 or even 4 of them.
So if optical interface will not be prohibitely expensive they will fairly soon replace soldered memories in AI oriented advanced hardware.
Entry level accelerators still would have relatively small amount and soldered wired memories.
3090s are still plently in use (heck, I have 2 myself), and A100s are still widely used 4 years after their launch. There's no decoupled solution that provides the same bandwidth that soldered memory does, which is of utmost importance for something like LLM, which are really bandwidth-bound. Mind providing any lead on such kind of offering? Current interconnects are the major bottlenecks in all clustered systems. Just saying "optical interface" doesn't mean much, since the current solutions are ate least one order of magnitude behind our soldered interfaces. Something like a 5090 would fit in this. It's considered an entry level accelerator for all purposes. The term "gpu-poor" is a good example of that.
I can see the point of your idea, but is not something that will take place at all within the next 5 years, and may take 10 years or more to become feasible. One pretty clear example of that is PCIe, with the current version 5.0 being a major bottleneck still, version 6.0 only coming to market next year, and 7.0 having its spec finished, but still way behind the likes of NVLink (PCIe 7.0 bandwidth will be somewhere between NVLink 2.0~3.0, which were Volta/Ampere links).
I believe NVLink is the fastest in-node interconnect in use in the market at the moment, and even it is still a bottleneck compared to the actual GPU memory.