Thursday, October 17th 2024
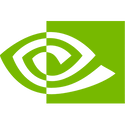
NVIDIA Fine-Tunes Llama3.1 Model to Beat GPT-4o and Claude 3.5 Sonnet with Only 70 Billion Parameters
NVIDIA has officially released its Llama-3.1-Nemotron-70B-Instruct model. Based on META's Llama3.1 70B, the Nemotron model is a large language model customized by NVIDIA in order to improve the helpfulness of LLM-generated responses. NVIDIA uses fine-tuning structured data to steer the model and allow it to generate more helpful responses. With only 70 billion parameters, the model is punching far above its weight class. The company claims that the model is beating the current top models from leading labs like OpenAI's GPT-4o and Antrophic's Claude 3.5 Sonnet, which are the current leaders across AI benchmarks. In evaluations such as Arena Hard, the NVIDIA Llama3.1 Nemotron 70B is scoring 85 points, while GPT-4o and Sonnet 3.5 score 79.3 and 79.2, respectively. Other benchmarks like AlpacaEval and MT-Bench spot NVIDIA also hold the top spot, with 57.6 and 8.98 scores earned. Claude and GPT reach 52.4 / 8.81 and 57.5 / 8.74, just below Nemotron.
This language model underwent training using reinforcement learning from human feedback (RLHF), specifically employing the REINFORCE algorithm. The process involved a reward model based on a large language model architecture and custom preference prompts designed to guide the model's behavior. The training began with a pre-existing instruction-tuned language model as the starting point. It was trained on Llama-3.1-Nemotron-70B-Reward and HelpSteer2-Preference prompts on a Llama-3.1-70B-Instruct model as the initial policy. Running the model locally requires either four 40 GB or two 80 GB VRAM GPUs and 150 GB of free disk space. We managed to take it for a spin on NVIDIA's website to say hello to TechPowerUp readers. The model also passes the infamous "strawberry" test, where it has to count the number of specific letters in a word, however, it appears that it was part of the fine-tuning data as it fails the next test, shown in the image below.
Sources:
NVIDIA, HuggingFace
This language model underwent training using reinforcement learning from human feedback (RLHF), specifically employing the REINFORCE algorithm. The process involved a reward model based on a large language model architecture and custom preference prompts designed to guide the model's behavior. The training began with a pre-existing instruction-tuned language model as the starting point. It was trained on Llama-3.1-Nemotron-70B-Reward and HelpSteer2-Preference prompts on a Llama-3.1-70B-Instruct model as the initial policy. Running the model locally requires either four 40 GB or two 80 GB VRAM GPUs and 150 GB of free disk space. We managed to take it for a spin on NVIDIA's website to say hello to TechPowerUp readers. The model also passes the infamous "strawberry" test, where it has to count the number of specific letters in a word, however, it appears that it was part of the fine-tuning data as it fails the next test, shown in the image below.
24 Comments on NVIDIA Fine-Tunes Llama3.1 Model to Beat GPT-4o and Claude 3.5 Sonnet with Only 70 Billion Parameters
65B-70B model size had been where things start to get really interesting, and the largest model a good home PC could locally run without excessively damaging quantization. They can be fun to play around with, and put quite a few things into perspective.
Yep, it works fine.
I was taught in school that using longer sentences than necessary to convey meaning lowers the quality of any scientific work. Just sayin'. This is exactly why AI is useless in the hands of critical thinkers, and dangerous in the hands of others.
1. It is trained by unreliable humans, and
2. The more LLM-generated answers form the basis of future answers, the more errors any new answer will produce.
Some can be found here: www.reddit.com/r/LocalLLaMA/
Edit: this one right here.
If the 5090 really comes with 32GB, those 64GB with a pair will be reall awesome.
It is somewhere no one had gone before until very recently, too; I don't think many could do that kind of roleplay with the level of responsiveness, variety, and apparent quality a GPU-hosted roleplay-finetuned LLM would provide. Play it like a holodeck, or even play it like the Matrix where you controlled everything. Yep, could see the appeal there.
But then again, many of the roleplay finetunes are also quite excellent for light scene writing and bouncing ideas off of and see how it sticks. They are already fit for use where no mathematics-level correctness nor absolute fidelity is required.
For that matter they are good for saving developer time too - sometimes all you need is a pointer to the right direction, and LLMs are good for that, even if the specific details might not be right.
What is implied but not explicitly stated is that whittling down LLaMa 3.1 to a 70 billion parameter model means that Nvidia improved on performance-per-watt and performance-per-dollar metrics by reducing the hardware requirements necessary to run an AI chatbot that compares favorably to GPT-4o and Claude 3.5 Sonnet.
Why is this important? One achievement that many are striving to attain is putting a useful model on a handheld device like a smartphone. I don't know if these model sizes scale linearly but perhaps a 8-10 billion parameter model could fit on a modern premium smartphone (8GB RAM).
AI adoption will take off once it runs well (and practically) on a smartphone, the primary computing modality of consumers in 2024.
Running an LLM AI chatbot on some PC loaded with two 24GB GPUs or pumped up with 128GB system RAM is not where consumer innovation is going to happen. It will happen in the handheld sector.
Getting more than 2 GPUs would be hard for me since I'm on a consumer platform that is limited in lanes/slots :(
Using an used/older server/WS platform would consume way too much power and be lacking in CPU perf, not to say the space required.
Doing wack jobs for splitting a single x8 slot into x4/x4 for more GPUs could be an option, but then the bandwidth limitation for training would still be annoying (not so much of an issue for inference tho).
But that's pretty much a rich-people-problem situation anyway haha This is talking about LLMs (such as what's running behind ChatGPT), and how Nvidia created a model based on Meta's Llama (which is open source) with way less parameters than what's usual, but with amazing performance.
An 8 Billion parameter model in 4-bit (0.5 bytes per parameter) would take about 4 GB of memory, then additional memory on top of that for processing text/requests/chatting, but 8GB should be sufficient. The biggest limitation would probably be the bandwidth of even fast LPDDR5X memory (the entirety of the model weights must be read for every token generated, although the maximum theoretical speed for a 64-bit LPDDR5X-8400 module iterating over a 4GB LLM would be acceptable—roughly 17 tokens/s), then possibly power consumption.