Friday, March 6th 2020
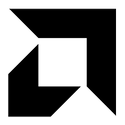
AMD Announces the CDNA and CDNA2 Compute GPU Architectures
AMD at its 2020 Financial Analyst Day event unveiled its upcoming CDNA GPU-based compute accelerator architecture. CDNA will complement the company's graphics-oriented RDNA architecture. While RDNA powers the company's Radeon Pro and Radeon RX client- and enterprise graphics products, CDNA will power compute accelerators such as Radeon Instinct, etc. AMD is having to fork its graphics IP to RDNA and CDNA due to what it described as market-based product differentiation.
Data centers and HPCs using Radeon Instinct accelerators have no use for the GPU's actual graphics rendering capabilities. And so, at a silicon level, AMD is removing the raster graphics hardware, the display and multimedia engines, and other associated components that otherwise take up significant amounts of die area. In their place, AMD is adding fixed-function tensor compute hardware, similar to the tensor cores on certain NVIDIA GPUs.AMD also talked about giving its compute GPUs advanced HBM2e memory interfaces, Infinity Fabric interconnect in addition to PCIe, etc. The company detailed a brief roadmap of CDNA looking as far into the future as 2021-22. The company's current-generation compute accelerators are based on the dated "Vega" architectures, and are essentially reconfigured "Vega 20" GPUs that lack tensor hardware.
Later this year, the company will introduce its first CDNA GPU based on "7 nm" process, compute unit IPC rivaling RDNA, and tensor hardware that accelerates AI DNN building and training.
Somewhere between 2021 and 2022, AMD will introduce its updated CDNA2 architecture based on an "advanced process" that AMD hasn't finalized yet. The company is fairly confident that "Zen4" CPU microarchitecture will leverage 5 nm, but hasn't been clear about the same for CDNA2 (both launch around the same time). Besides ramping up IPC, compute units, and other things, the design focus with CDNA2 will be hyper-scalability (the ability to scale GPUs across vast memory pools spanning thousands of nodes). AMD will leverage its 3rd generation Infinity Fabric interconnect and cache-coherent unified memory to accomplish this.
Much like Intel's Compute eXpress Link (CXL) and PCI-Express gen 5.0, Infinity Fabric 3.0 will support shared memory pools between CPUs and GPUs, enabling scalability of the kind required by exascale supercomputers such as the US-DoE's upcoming "El Capitan" and "Frontier." Cache coherent unified memory reduces unnecessary data-transfers between the CPU-attached DRAM memory and the GPU-attached HBM. CPU cores will be able to directly process various serial-compute stages of a GPU compute operation by directly talking to the GPU-attached HBM and not pulling data to its own main memory. This greatly reduces I/O stress. "El Capitan" is an "all-AMD" supercomputer with up to 2 exaflops (that's 2,000 petaflops or 2 million TFLOPs) peak throughput. It combines AMD EPYC "Genoa" CPUs based on the "Zen4" microarchitecture, with GPUs likely based on CDNA2, and Infinity Fabric 3.0 handling I/O.
Oh the software side of things, AMD's latest ROCm open-source software infrastructure will bring CDNA and CPUs together, by providing a unified programming model rivaling Intel's OneAPI and NVIDIA CUDA. A platform-agnostic API compatible with any GPU will be combined with a CUDA to HIP translation layer.
Data centers and HPCs using Radeon Instinct accelerators have no use for the GPU's actual graphics rendering capabilities. And so, at a silicon level, AMD is removing the raster graphics hardware, the display and multimedia engines, and other associated components that otherwise take up significant amounts of die area. In their place, AMD is adding fixed-function tensor compute hardware, similar to the tensor cores on certain NVIDIA GPUs.AMD also talked about giving its compute GPUs advanced HBM2e memory interfaces, Infinity Fabric interconnect in addition to PCIe, etc. The company detailed a brief roadmap of CDNA looking as far into the future as 2021-22. The company's current-generation compute accelerators are based on the dated "Vega" architectures, and are essentially reconfigured "Vega 20" GPUs that lack tensor hardware.
Later this year, the company will introduce its first CDNA GPU based on "7 nm" process, compute unit IPC rivaling RDNA, and tensor hardware that accelerates AI DNN building and training.
Somewhere between 2021 and 2022, AMD will introduce its updated CDNA2 architecture based on an "advanced process" that AMD hasn't finalized yet. The company is fairly confident that "Zen4" CPU microarchitecture will leverage 5 nm, but hasn't been clear about the same for CDNA2 (both launch around the same time). Besides ramping up IPC, compute units, and other things, the design focus with CDNA2 will be hyper-scalability (the ability to scale GPUs across vast memory pools spanning thousands of nodes). AMD will leverage its 3rd generation Infinity Fabric interconnect and cache-coherent unified memory to accomplish this.
Much like Intel's Compute eXpress Link (CXL) and PCI-Express gen 5.0, Infinity Fabric 3.0 will support shared memory pools between CPUs and GPUs, enabling scalability of the kind required by exascale supercomputers such as the US-DoE's upcoming "El Capitan" and "Frontier." Cache coherent unified memory reduces unnecessary data-transfers between the CPU-attached DRAM memory and the GPU-attached HBM. CPU cores will be able to directly process various serial-compute stages of a GPU compute operation by directly talking to the GPU-attached HBM and not pulling data to its own main memory. This greatly reduces I/O stress. "El Capitan" is an "all-AMD" supercomputer with up to 2 exaflops (that's 2,000 petaflops or 2 million TFLOPs) peak throughput. It combines AMD EPYC "Genoa" CPUs based on the "Zen4" microarchitecture, with GPUs likely based on CDNA2, and Infinity Fabric 3.0 handling I/O.
Oh the software side of things, AMD's latest ROCm open-source software infrastructure will bring CDNA and CPUs together, by providing a unified programming model rivaling Intel's OneAPI and NVIDIA CUDA. A platform-agnostic API compatible with any GPU will be combined with a CUDA to HIP translation layer.
30 Comments on AMD Announces the CDNA and CDNA2 Compute GPU Architectures
/s
7N+, on the other hand, looks like a waste, if what TSMC is promisnig about 5nm is true. I thought AMD having enough money to have separate line of compute oriented products was good news for gamers.
Did what?
Looking forward though consider, Any benchmark or rumour on a video output enabled big Navi is not CDNA.
Spec sheet rumours could be either too.
Key differences...
NV locks you into an eco system. AMD hasn't made a CUDA or done everything they can to force you to only use Radeons for things like 'Physx' and threatened to sue people who dare to undo their locks.
AMD is actually trying to make this easy to use and support open standards while trying to ease the pain of moving from the green tax team.
I also very muchly doubt we'll see the end of products like the Vega 2s, because there's a purpose for render/compute beast cards.
Though who knows, Quadros are nothing but really expensive GeForce cards with 'pro' drivers in most cases.
AMD on the other hand lets you use their Pro drivers on their normal Radeons. The difference is the real pro cards tend to have better FP64 dividers.
Sooooo...
How's AMD being greedy again?
ROCm is also an open-source framework. It is also C++ code that can run on any hardware too. There are some AMD-only extensions that are specific to GCN5 (and RDNA as of 3.0 now) but they are mostly raw OpenCL, which can be run on NVIDIA GPUs, Intel IGPs and even x86/ARM CPUs using standard LLVM compilers. They are also Metal (Apple) compatible, considering that Macs are using AMD GPUs for the Pro products.
Whats the problem? There isn't any! What it is is that it's more of a limitation because coding pure (without extensions and optimizations) OpenCL is not as robust as CUDA on NVIDIA GPGPUs. It's great for initial learning and early development, but eventually once you get to optimization, you'd want a framework that can handle complex loads and support for optimizing further.
HIP makes it easy to use CUDA, HC, OpenCL, etc. code as it translates quite cleanly. So any code compiled with HIP enabled (using HCC on AMD, NVCC on NVIDIA) will work on any GPGPU.
NVIDIA has not made CUDA proprietary. It is only a "walled garden" because CUDA-optimized code runs better on NVIDIA hardware, and this is obviously sensible. If you translate CUDA code using HIP, you can run it on AMD GPUGPUs, but don't expect it to be optimized since the underlying architecture is different. OpenCL runs fine on any hardware, hence being open, only being limited by the hardware.
I actually went digging. AMD can only partially emulate some CUDA code. They can only do it on Linux. They are also versions behind.
NV did offer an emulator for a bit, but then killed it and locked the CUDA dev tools to only run on certified hardware.
If AMD ever did try to actually license CUDA, NV would have to change the license. You require Nvidia hardware, you require Nvidia drivers, and God help you if you try to modify their software.
So basically AMD can't even look at CUDA while trying to port it.
Open and free as long as you only use our hardware. Oh you could use OpenCL but it's badly supported and very limited, but here use our 'Free' and superior 'open' alternative.
Saying it's not a walled garden is like saying OSX isn't because it's based on FreeBSD. Apple keeps making it harder and harder to run on non-Apple hardware.
NV has a stack of clauses forbidding people from reverse engineering anything they provide in terms of CUDA or it's required software it seems.
Just because people have managed to hack support for some CUDA calls to work in OpenCL or One API, doesn't mean it's open. Oh and you are completely screwed on Windows.
This is what I gathered in a few minutes of Google searches.
Nvidia won't even let you decompile the CUDA stuff on Linux. The license actually says you are only allowed to decompress the downloaded file, and distribute the unzipped contents. Any other modification or tampering or unpacking will be viewed most dimly.
So it's impressive that people have managed to get any CUDA porting to work. Though I'm sure if NV could prove that they looked at actual CUDA stuff they'd sue their asses into non-existance.
Edit: from what I gathered...
ROCm is an attempt by AMD to emulate CUDA hardware.
HIPM is software that translates CUDA code into OpenCL code.
Neither offers full support for even older versions of CUDA and they don't support the latest versions at all.
Also no CUDA emulators on Windows or NV will sue you into bankruptcy. Guess they really don't want PhysX on anything but NV hardware that is also rendering. No slave NV GPUs with Radeons doing the drawing.
Open like iOS... LoL
Hot tip: never listen to fanbois. They have no real information due to their inherent cognitive bias. Wow that is plain uninformed, if not disingenuous.
CUDA was locked down prior to 2012 and could only run on NVIDIA GPGPUs as there was practically no competition. OpenCL at the time (1.2) was not as useful for compute until 2.0 (due to C11 and piping support). This is why CUDA has a lead in deep learning implementation today.
OpenCL is definitely not badly supported by NVIDIA. In fact most implementations of it run fine on both NVIDIA and AMD GPGPUs. It's just that implementations that use CUDA code have a specific use case and have proven to be more efficient than trying to implement it in vanilla OpenCL.
Are you arguing about open-source solutions or the superiority of one API over another? Because despite CUDA being proprietary (in terms of hardware efficiency), NVIDIA hasn't done anything wrong since it is their product.
ROCm is a very new (as in less than 2 years old) framework that I very much want to succeed, but direct support (from AMD) is lacking. This is expected as it is an open-source solution and AMD is a smaller company that most likely doesn't have enough resources to send help out. This is where NVIDIA (and Intel apparently) shines. Hopefully the funding from the US DOE will accelerate its development as a viable framework.
Dedicated hardware vs a jack all trades and a master of none. Makes more sense.
Seems they are available for all GCN cards. Which is really kind of cool. Those certified drivers aren't cheap to produce and have certified.