Thursday, May 14th 2020
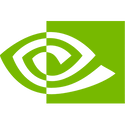
NVIDIA GA100 Scalar Processor Specs Sheet Released
NVIDIA today unveiled the GTC 2020, online event, and the centerpiece of it all is the GA100 scalar processor GPU, which debuts the "Ampere" graphics architecture. Sifting through a mountain of content, we finally found the slide that matters the most - the specifications sheet of GA100. The GA100 is a multi-chip module that has the 7 nm GPU die at the center, and six HBM2E memory stacks at its either side. The GPU die is built on the TSMC N7P 7 nm silicon fabrication process, measures 826 mm², and packing an unfathomable 54 billion transistors - and we're not even counting the transistors on the HBM2E stacks of the interposer.
The GA100 packs 6,912 FP32 CUDA cores, and independent 3,456 FP64 (double-precision) CUDA cores. It has 432 third-generation tensor cores that have FP64 capability. The three are spread across a gargantuan 108 streaming multiprocessors. The GPU has 40 GB of total memory, across a 6144-bit wide HBM2E memory interface, and 1.6 TB/s total memory bandwidth. It has two interconnects: a PCI-Express 4.0 x16 (64 GB/s), and an NVLink interconnect (600 GB/s). Compute throughput values are mind-blowing: 19.5 TFLOPs classic FP32, 9.7 TFLOPs classic FP64, and 19.5 TFLOPs tensor cores; TF32 156 TFLOPs single-precision (312 TFLOPs with neural net sparsity enabled); 312 TFLOPs BFLOAT16 throughout (doubled with sparsity enabled); 312 TFLOPs FP16; 624 TOPs INT8, and 1,248 TOPS INT4. The GPU has a typical power draw of 400 W in the SXM form-factor. We also found the architecture diagram that reveals GA100 to be two almost-independent GPUs placed on a single slab of silicon. We also have our first view of the "Ampere" streaming multiprocessor with its FP32 and FP64 CUDA cores, and 3rd gen tensor cores. The GeForce version of this SM could feature 2nd gen RT cores.
The GA100 packs 6,912 FP32 CUDA cores, and independent 3,456 FP64 (double-precision) CUDA cores. It has 432 third-generation tensor cores that have FP64 capability. The three are spread across a gargantuan 108 streaming multiprocessors. The GPU has 40 GB of total memory, across a 6144-bit wide HBM2E memory interface, and 1.6 TB/s total memory bandwidth. It has two interconnects: a PCI-Express 4.0 x16 (64 GB/s), and an NVLink interconnect (600 GB/s). Compute throughput values are mind-blowing: 19.5 TFLOPs classic FP32, 9.7 TFLOPs classic FP64, and 19.5 TFLOPs tensor cores; TF32 156 TFLOPs single-precision (312 TFLOPs with neural net sparsity enabled); 312 TFLOPs BFLOAT16 throughout (doubled with sparsity enabled); 312 TFLOPs FP16; 624 TOPs INT8, and 1,248 TOPS INT4. The GPU has a typical power draw of 400 W in the SXM form-factor. We also found the architecture diagram that reveals GA100 to be two almost-independent GPUs placed on a single slab of silicon. We also have our first view of the "Ampere" streaming multiprocessor with its FP32 and FP64 CUDA cores, and 3rd gen tensor cores. The GeForce version of this SM could feature 2nd gen RT cores.
101 Comments on NVIDIA GA100 Scalar Processor Specs Sheet Released
You're comparing orange to apples. You can't use different architectures as basis... Do I really need to explain the density difference between Volta at 12nm (24mT/mm²) vs Ampere at 7nm (65mT/mm²) ?
I pity you that you pity me :).
But you didn't answer, what are you still doing here ? Let the case rest buddy, you said it's settled. Feeling insecure about the nonsense that you wrote ?
Tenor cores are now compliant with accelerating IEEE-compliant FP64 computations
Each FP64 matrix multiply add op now replaces 8 FMA FP64 operation
Meaning each SM is now capable of 128 FP64 op per clock which achieves 2.5X the throughput of V100 I am here simply to educate. There is no typo, they did the same on this official slide:
Don't worry, just as you can spam the same incorrect statements a million times I can also correct you every time. I did not need or request your worthless education. I mean for one thing you are absolutely clueless, who do you think you are, a scholar ? On some random tech forum wasting your time spamming the same shit over and over ?
Wake up to the real world buddy, you ain't educating anyone. :roll:
www.nextplatform.com/2020/05/14/nvidia-unifies-ai-compute-with-ampere-gpu/
Sucks to be you I guess.
As already mentioned, the A100 is 2.5x more efficient in accelerating FP64 workloads compared to the V100. This was achieved by replacing the traditional DFMA instructions with FP64 based matrix multiply-add. This reduces the scheduling overhead and shared memory bandwidth requirement by cutting down on instruction fetches.
www.hardwaretimes.com/nvidia-ampere-architectural-analysis-a-look-at-the-a100-tensor-core-gpu/amp/
With FP64 and other new features, the A100 GPUs based on the NVIDIA Ampere architecture become a flexible platform for simulations, as well as AI inference and training — the entire workflow for modern HPC. That capability will drive developers to migrate simulation codes to the A100.
Users can call new CUDA-X libraries to access FP64 acceleration in the A100. Under the hood, these GPUs are packed with third-generation Tensor Cores that support DMMA, a new mode that accelerates double-precision matrix multiply-accumulate operations.
A single DMMA job uses one computer instruction to replace eight traditional FP64 instructions. As a result, the A100 crunches FP64 math faster than other chips with less work, saving not only time and power but precious memory and I/O bandwidth as well.
We refer to this new capability as Double-Precision Tensor Cores.
agenparl.eu/double-precision-tensor-cores-speed-high-performance-computing/
Ouch, suck for you again!
Imagine this, everything you post agrees with me not with you. Sucks to be you I guess !
By the way, can we like schedule these. Like for instance, let's post one comment every half an hour or something ?
And in fact the GA100 is extemely efficient in what it was designed for - AI training/inference. GV100 only supports accelerated tensor operations for the FP16 format, so that is the best base comparison, comparing tensor operations on GV100 with tensor operations on the GA100. All the other types of operations on a GV100, like FP32, INT8, etc. fall back to general compute units (they are not accelerated by Tensor Cores). FP16 performance of a GV100 is 125 TOPS. For a GA100, that is 310 TOPS baseline (2.5x better), 625 TOPS (5x better) with the sparse feature on (but that is a logical optimization, not raw performance). So we have 125 TOPS for GV100 at 250W and 310TOPS for GA100 at 400W. With basic math skills you can easily see that Ampere's energy efficiency (performance per watt) is actually 55% better compared to GV100. That's raw FMA performance. With the optimization features on, it can actually reach up to 200% better energy efficiency.
I can understand why AMD fanboys like Vya Domus are bitter. AMD's "AI accelerators" offer only a tiny fraction of performance compared to Ampere. They are not really AI accelerators - you can get orders of magnitude better performance from hardware from Google, Nvidia and other companies. Can you run AI training/inferencing on the AMD cards? Yes, but you can do that on any x86 CPU as well. Would doing so on an AMD card make any sense? No, just like it doesn't make sense on a CPU anymore. Fixed function hardware like Nvidia's Tensor Cores on the GA100 or Google's Tensor Processing Unit are way better for this task.
Also Vya Domus, unfortunately for you MuhammedAbdo is generally correct. Nvidia compares the FP32 tensor performance on Ampere with FP32 non-tensor performance on Volta, simply because Volta does not support FP32 tensor operations (falls back to general compute units) and Ampere does. The only issue with Muhammed's statement is that he also reverted the implication backwards, which is of course incorrect. Tensor Cores cannot perform the full set of FP32 operations that a general compute unit can. However the rest of your statements, Vya Domus, are incorrect and show that you have very little understanding of the technology.
Also, nice new account with posts only about calling people AMD fanboys bro. Welcome to TPU :).
As for your "that was my only statement", let me recap: Here you're missing the fact that GA100 is focused entirely on AI (it even has it in the full name - Nvidia A100 Tensor Core GPU) and it's performance has nothing to do with how games are going to perform on other Ampere chips. Here you fail to understand that the large chips are actually designed with fabrication errors in mind from the start and where you miss that the 400W TDP still translates to 55% increase in energy efficiency compared to Volta. By actually measuring the performance and the dividing that by power consumption. As has been done here on TPU for years. But you're the expert. You tell the big boss here that all his reviews were wrong and that he should've inferred efficiency from SM counts and all those perf/power measurements were useless. Go ahead. Looking at Pascal vs Polaris/Vega/GCN in general - Pascal with much smaller chips and much higher frequencies did have lower power consumption.
Vega 64 balanced with standard BIOS @ 1274 MHz - 292W, 1080Ti standard @ 1481 MHz 231W
www.techpowerup.com/review/amd-radeon-rx-vega-64/29.html
While at the same time 1080ti has +30% to +40% more performance, 1080ti is a 471 mm² chip at 16nm, while Vega 64 is a 486 mm² chip at 14nm. AMD = larger, slower, power hungry. And it's been the same story throughout the Polaris/Vega/Maxwell/Pascal/Turing generations. So I'm curious - what kind of data are you basing your statement on? Here you are comparing general compute unit performance, ignoring the fact the GA100 chip design invested heavily into fixed function units (tensor cores) and actually achieves 2.5x raw performance increase, with +55% energy efficiency compared to Volta. This is perhaps the most startling showcase of how you have no clue what you're talking about. Of course it is possible to do tensor operations on general compute units (SMs). And in fact that is what Volta was doing for anything else besides FP16 tensor ops and it is what even AMD GPUs are doing. Radeons do not have tensor cores, yet it's no problem to run let's say Google's TensorFlow on that hardware. Why? 3D graphics is actually mostly about matrix and vector multiplications, dot products etc., so general compute units are quite good at it - much better than CPUs, not as good as fixed function units like Tensor Cores. It's very obvious to anyone by now that you have not. You are lacking the very essentials required to do that.
To quote your own evaluation of the other guy, "I am convinced you can't be educated, you are missing both the will and the capacity to understand this." It fits you better that it fits him.
This means it's totally reasonable to look at this chip and infer future performance in a consumer GPU. The number of SMs , clock speeds, power envelope will vary but the architecture wont. Of course if you don't know much it's going to seem like you can't extrapolate performance, that's not surprising. 18% of the shaders are disabled, that's a huge amount, that's not meant to improve redundancy. You add one, maybe two SMs for that not 20 almost a fifth of the total SMs, they made a chip too large to be viable fully enabled on this current node. Don't be a bitter fanboy and look at things objectively and pragmatically.
V100 which was almost as large was fully enabled from day one, guess that one never had any fabrication errors right ? Nah, more like your explanation is just wrong. Just raw FP32 performance. You could factor in FP16/FP64 performance and then the Pascal equivalents would look orders of magnitude less efficient. But none of that matters because I was speaking purely from the perspective of how ICs behave, power increases linearly with frequency but voltage is squared. Therefor as a general rule a chip twice as large but running at half the frequency (and therefor it would require lower voltage) would be more efficient simply by matters of physics, maybe this was too complicated for you too understand. Don't push your self too hard. OK fanboy. That's what this is all about, isn't it ? You're just a bitter Nvidia fanboy that has nothing better to do, you don't want to discuss anything, you just want to bash a brand. That's sad and pathetic. It achieves 2.5X more performance and 55% better efficiency in some workloads, not all. You're just starting to regurgitate the same stuff over and over, a lot like your friend. Well, I am fairly convinced this is just an alt account. Hi there buddy. What's startling is that even though you're trying to scour through my old comments like some creepy detective wanna be, I made myself very clear that those units are general purpose and can execute any sort of code. It's obvious I was referring to native tensor ops using hardware, but you are so caught up in your bitter fanboy rampage you are desperately trying to find anything to quote me on. Sad, really fucking sad. Try me, or you're too scared of showing us how little you know ? Don't be, you've already shown that, might as well go all in. "him", riiiiight
Fact is you really have no clue do you? Only a rabid AMD fanboy would focus on traditional FP32 for an AI chip, especially when the new TF32 format is 20 times higher than previous gen.
And only a rabid AMD fanboy would lack the imagination that NVIDIA will cut tensor core count to a 1/4 (as they are now miles faster than before), cut the HPC stuff out, remove NVLink, clock the chip higher and achieve a solid gaming GPU with at least 50% power efficiency than previous gen and the competition (it's already higher than 50% effeciency, 54 bilion transistor running 400w, compared to 10 billion in 5700XT running 225w)!
Is someone making you do this at gun point ? Should we inform the authorities ? Write us an SOS message or something.
The key is efficiency here. Efficiency is about how well the GPU scheduler can deliver work to the existing resources of a GPU, in other words how well it can keep the resources busy. And it's not a choice on AMD's side to over-provision the compute cores (your very theoretical "raw performance"). Their scheduler architectures are inferior to Nvidia's, so they must provide more compute units in order to compete, since they cannot keep them all busy and therefore lack efficiency. They also do the same with clock frequencies. Polaris/Vega were designed for much lower optimal frequencies (perf/power), but because of the leaps in performance Maxwell/Pascal made, AMD had to set the core clocks on their Polaris/Vega/RDNA architectures way past the optimal point. Again the reason is to remain at least a little bit competitive. That's the main reason for the terrible power efficiency AMD has. And even with RDNA it's still there. It's just hidden by the 7nm node improvements. Let's not forget that 7nm RDNA GPUs are barely catching up to 12nm Turing chips. Just the process difference alone is almost 4 times the MTMM. Efficiency is simply not there, even with RDNA. We'll see that with Ampere gaming GPUs. Then we'll have a reasonable comparison (almost, since the RDNA GPUs lack raytracing and many other features). Looks like my original remark hurt you more than I expected. Good that you are trying to repeat it. Imitation is the highest form of flattery. It achieves 2.5x more performance and 55% better efficiency in workloads for which it was designed. What you're trying to do, and trust me that everyone here does see your funny attempt, is evaluating a car based on how well it can fly, then trying to say it's not a good car, because it doesn't fly well. (although amusingly in this case even at flying the "car" would still perform much better than competition's best attempt, e.g. the GA100 classic compute is still much better than that of AMD compute cards) Hey, don't be butthurt about saying you never made any other statements and then being slapped in the face with said "non-existent" statements with gusto. And your statement regading the alleged inability of general compute units to execute tensor ops is quite clear. Let me repost it here: How does "they just do scalar ops" mean "can execute any sort of code"? Hilarious. Please do. I intentionally ommited that in my original reply, because you sir rule and deserve a quote!
It's good info, but the barbs just sully the conversation. :(
In shipping form, radeon VII and Radeon mi50 do not come fully enabled(6% disabled), only increase fp32 while moving to 7nm by 9% while using the same power vs Vega 64(a inefficient chip to begin with). In addition, Vega 20 does not represent remotely as ambitious a leap as Nvidia as A100 as it less than 1 half the size and about a quarter of the amount of transistors.
One more reason why Nvidia has to disable quite a bit of the chip is pure volume.
Do you know how much Nvidia Data center + professional visualization revenue is? Last Quarter it was 1.3 billion dollars. That is near the revenue of AMD's CPU and graphic division which produced 1.43 billion dollars for q1. Nvidia financials tomorrow will likely produce a figure that is equal to this value.
Considering this, Nvidia must deliver enormous volume which means that yields have to take a hit to deliver to their customers. As a result, Nvidia yields for these chips have to suffer to deliver the volume wanted by their customers and this will continue be a problem in the future.
Analysts are predicting Nvidia's data center revenue to grow from 5.5 billion annually to 20 billion which is the revised prediction after A100 was released. Nvidia's market capitalization is close to Intel's right now. The reason being that the data center market is growing at an explosive speed and products from Intel and AMD are not perceived a threat in the near future(analysts already know what next gen AMD and Intel products look like as they are well connected). From the tone of your post, it seems you perceive A100 as a failure but your own bias is blurring your vision to what should be obvious. Look at the reaction from the markets(NVDA stock has grown 15% since A100 release and analysts have revised Nvidia stock value target from $275 to $420), the tangible benefits of A100 to the data center market and you will realize how blind you were to the success of this product. Scoffing off a100 prowess is just showing your own ignorance.
How compelling ampere will be is difficult to say. I do like that DLSS is improving and becoming more flexible though it's still quite developer dependent it doesn't just work with everything so while it's a great option to have when available it's of no real use otherwise. I'm a much bigger fan of performance or image quality enhancements I can just outright utilize whenever without being reliant on a developer to implement it's functionality. We've seen how well that works with mGPU for CF/SLI I mean hey developers are "lazy" or more appropriately from their own perspective time is money. A little bit of a AMD STANboi...on my Intel CPU & Nvidia GPU...is what it is I did quite like AMD's CPU's prior to bulldozer and it's current CPU from the release Ryzen have been highly "compelling" even if they weren't 100% perfect and continued to extend upon that aspect. They've even managed to make Intel's products drastically more compelling than the dogsh*t products they'd been overpricing and selling to consumers taking advantage of everyone they could.